Flexible deep learning network model compression method based on channel gradient pruning
A channel and gradient technology, applied in the field of flexible deep learning network model compression, can solve the problems of inability to predict the number of floating-point operations per second, and cannot directly reflect the actual compression rate, so as to improve the accuracy of pruning and strong predictability Effect
- Summary
- Abstract
- Description
- Claims
- Application Information
AI Technical Summary
Problems solved by technology
Method used
Image
Examples
Embodiment Construction
[0029] In order to understand the purpose, features and advantages of the present invention more clearly, the technical solutions of the present invention will be described in detail below in conjunction with the accompanying drawings and specific embodiments.
[0030] The present invention is a flexible deep learning network model compression method based on channel gradient pruning, the specific process is as follows:
[0031] Step 1: Obtain the deep convolutional neural network model to be pruned
[0032] Step 1-1: Add mask layer constraints to the convolutional layers that need to be pruned in the original deep convolutional neural network. Import the pre-trained weight parameters obtained from the network into the original deep convolutional neural network with added constraints to form a deep convolutional neural network model to be pruned. The number of channels in the masking layer corresponds to the number of channels in the convolutional layer considering pruning, a...
PUM
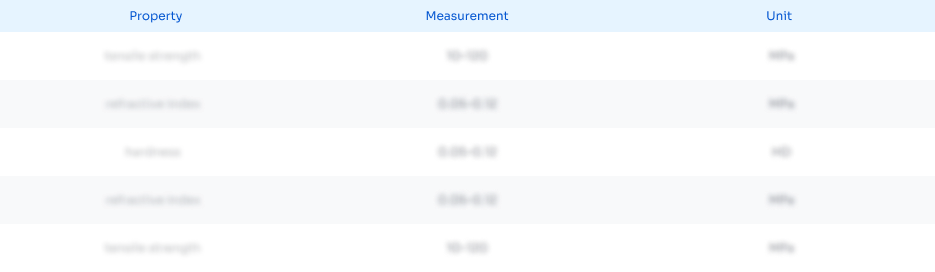
Abstract
Description
Claims
Application Information
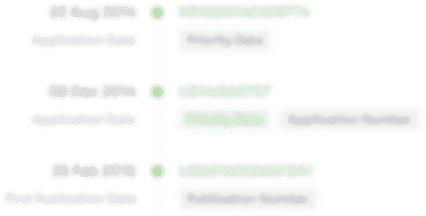
- R&D Engineer
- R&D Manager
- IP Professional
- Industry Leading Data Capabilities
- Powerful AI technology
- Patent DNA Extraction
Browse by: Latest US Patents, China's latest patents, Technical Efficacy Thesaurus, Application Domain, Technology Topic.
© 2024 PatSnap. All rights reserved.Legal|Privacy policy|Modern Slavery Act Transparency Statement|Sitemap