User side load probability prediction method based on time domain convolutional neural network
A convolutional neural network and probability prediction technology, which is applied in the field of user-side load probability prediction based on time-domain convolutional neural network, can solve problems such as model structural defects
- Summary
- Abstract
- Description
- Claims
- Application Information
AI Technical Summary
Problems solved by technology
Method used
Image
Examples
Embodiment
[0057] figure 1 It is a flow chart of the user side load probability prediction method based on the time domain convolutional neural network in the present invention.
[0058] In this example, if figure 1 As shown, the present invention is based on a time-domain convolutional neural network user side load probability prediction method, comprising the following steps:
[0059] S1. Data collection and preprocessing
[0060] S1.1. Collect historical load, temperature and weather data
[0061] In a certain time range, the user's active power is collected according to a fixed cycle as historical load data X load , and simultaneously collect the weather type X in the corresponding time range weather and temperature value X temperature , in this embodiment, the sampling period is 15 minutes, i.e. 96 points / day section; then the weather and temperature data are processed as data samples X=(X weather ,X temperature ,X load );
[0062] S1.2. Data sample cleaning
[0063] Perfo...
PUM
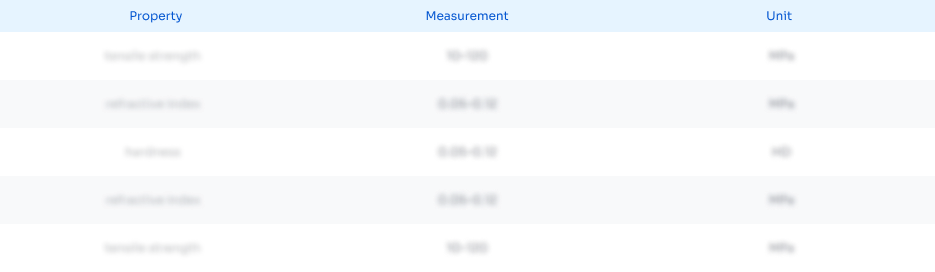
Abstract
Description
Claims
Application Information
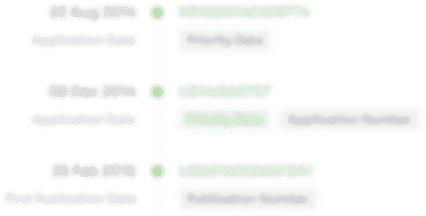
- R&D Engineer
- R&D Manager
- IP Professional
- Industry Leading Data Capabilities
- Powerful AI technology
- Patent DNA Extraction
Browse by: Latest US Patents, China's latest patents, Technical Efficacy Thesaurus, Application Domain, Technology Topic, Popular Technical Reports.
© 2024 PatSnap. All rights reserved.Legal|Privacy policy|Modern Slavery Act Transparency Statement|Sitemap|About US| Contact US: help@patsnap.com