Target detection algorithm based on wheat image
A target detection algorithm and detection algorithm technology, applied in computing, computer components, neural learning methods, etc., can solve problems such as small targets, few dataset samples, and unbalanced categories, and achieve the advantages of high inference speed, real-time inference, The effect of reducing requirements
- Summary
- Abstract
- Description
- Claims
- Application Information
AI Technical Summary
Problems solved by technology
Method used
Image
Examples
Embodiment 1
[0024] Embodiment 1: see figure 1 , a kind of target detection algorithm based on wheat image, described detection algorithm comprises the following steps:
[0025] Step 1: The first stage is the training stage of the algorithm,
[0026] Step 2: The second stage is the real-time reasoning stage of the algorithm.
[0027] Such as figure 1 As shown, this algorithm gives the training steps of the training phase:
[0028] Step S11, clustering the anchors of YOLOv5 using k-means. Add the width w and length h of all the boxes in the wheat training set label to the list to define the distance between the two boxes A and B The distance d is the similarity measurement method of the k-means algorithm, because the number of anchors in YOLOv5 is 9, so k=9 is used for clustering. Replace the original anchor of YOLOv5 with the obtained 9 new anchor values. Note: The larger the anchor, the deeper the layer, because the feature map of the deeper layer is responsible for detecting large...
PUM
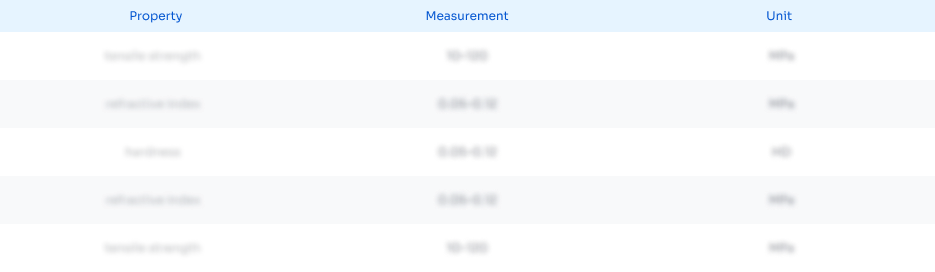
Abstract
Description
Claims
Application Information
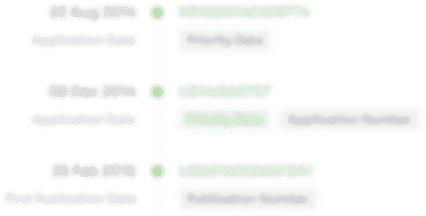
- R&D Engineer
- R&D Manager
- IP Professional
- Industry Leading Data Capabilities
- Powerful AI technology
- Patent DNA Extraction
Browse by: Latest US Patents, China's latest patents, Technical Efficacy Thesaurus, Application Domain, Technology Topic, Popular Technical Reports.
© 2024 PatSnap. All rights reserved.Legal|Privacy policy|Modern Slavery Act Transparency Statement|Sitemap|About US| Contact US: help@patsnap.com