Carrier roller fault diagnosis method and system based on machine learning and storage medium
A technology of fault diagnosis and machine learning, which is applied in the field of fault diagnosis of conveyor rollers, can solve problems such as the inability to quickly and efficiently judge roller faults, and achieve the effects of reducing impact, improving accuracy, and improving safety
- Summary
- Abstract
- Description
- Claims
- Application Information
AI Technical Summary
Problems solved by technology
Method used
Image
Examples
Embodiment 1
[0044] like figure 1 As shown, this embodiment provides a machine learning-based idler fault diagnosis method, including the following steps:
[0045] S1, collect the audio data of the roller;
[0046] S2, extracting features of the audio data; the features of the audio data specifically include one or any combination of sharpness, noise annoyance, and speech interference level;
[0047] S3, the characteristics of the audio data are input into the trained CART model, and the CART model recognizes the running state of the idler;
[0048] S4, if the idler is running abnormally, perform alarm, monitoring or control operations; if the idler is running normally, complete the fault diagnosis of the idler at the current moment, and perform step S5;
[0049] S5, update the time, repeatedly execute step S1 to step S4, and carry out idler fault diagnosis at the next time.
[0050]This embodiment uses voice signal processing technology to analyze the sound quality of the audio data of...
Embodiment 2
[0084] like Figure 4 As shown, this embodiment provides a machine learning-based idler fault diagnosis system, and the machine learning-based idler fault diagnosis system described below and the above-mentioned machine learning-based idler fault diagnosis method can be referred to.
[0085] see Figure 4 As shown, the system includes the following modules connected in sequence: data acquisition module, feature extraction module, CART model module and monitoring module;
[0086] In this embodiment, the data collection module is used to collect the audio data of the idler; the feature extraction module is used to extract the features of the audio data; the CART model module is used to identify the running state of the idler according to the features of the audio data; the monitoring module is used for Perform alert, monitor, or control actions based on the identification and classification results of the logistic regression model module. The CART model module stores the train...
Embodiment 3
[0091] Corresponding to the above method embodiment, this embodiment also provides a readable storage medium, a readable storage medium described below and a machine learning-based idler fault diagnosis method described above can refer to each other .
[0092] A readable storage medium, on which a computer program is stored, and when the computer program is executed by a processor, the steps of the machine learning-based idler fault diagnosis method of the above method embodiment are implemented.
[0093] Specifically, the readable storage medium may be a U disk, a mobile hard disk, a read-only memory (Read-Only Memory, ROM), a random access memory (Random Access Memory, RAM), a magnetic disk or an optical disk, and other storage devices that can store program codes. Read storage media.
PUM
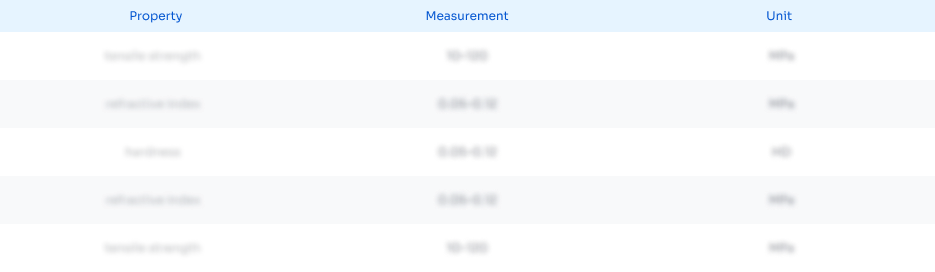
Abstract
Description
Claims
Application Information
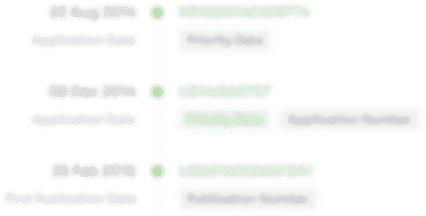
- R&D
- Intellectual Property
- Life Sciences
- Materials
- Tech Scout
- Unparalleled Data Quality
- Higher Quality Content
- 60% Fewer Hallucinations
Browse by: Latest US Patents, China's latest patents, Technical Efficacy Thesaurus, Application Domain, Technology Topic, Popular Technical Reports.
© 2025 PatSnap. All rights reserved.Legal|Privacy policy|Modern Slavery Act Transparency Statement|Sitemap|About US| Contact US: help@patsnap.com