Light field multi-view image super-resolution reconstruction method based on deep learning
A technology of super-resolution reconstruction and deep learning, applied in the field of light-field multi-view image super-resolution reconstruction based on deep learning, can solve the problem that the light-field multi-view image super-resolution method cannot meet the technical indicators, and achieve enhanced accuracy. Effect
- Summary
- Abstract
- Description
- Claims
- Application Information
AI Technical Summary
Problems solved by technology
Method used
Image
Examples
Embodiment Construction
[0021] According to one or more embodiments, such as figure 1 As shown, a light field multi-view image super-resolution method based on multi-scale fusion features includes the following steps:
[0022] A1, using light field camera multi-view images or light field camera array images (multi-view images distributed in an N×N array) to construct a training set of high-resolution and low-resolution image pairs;
[0023] A2, construct a multi-layer feature extraction network from the N×N light field multi-view image array to the N×N light field multi-view feature image;
[0024] A3, stack feature images and build feature fusion and enhanced multi-layer convolutional network to obtain 4D light field structural features that can be used to reconstruct light field multi-view images;
[0025] A4, build an upsampling module to obtain the nonlinear mapping relationship from 4D light field structural features to high-resolution N×N light field multi-view images;
[0026] A5, build a lo...
PUM
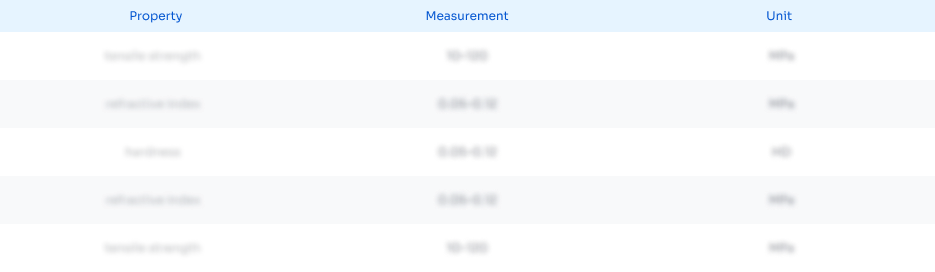
Abstract
Description
Claims
Application Information
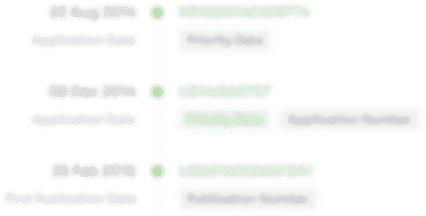
- R&D Engineer
- R&D Manager
- IP Professional
- Industry Leading Data Capabilities
- Powerful AI technology
- Patent DNA Extraction
Browse by: Latest US Patents, China's latest patents, Technical Efficacy Thesaurus, Application Domain, Technology Topic, Popular Technical Reports.
© 2024 PatSnap. All rights reserved.Legal|Privacy policy|Modern Slavery Act Transparency Statement|Sitemap|About US| Contact US: help@patsnap.com