Multi-scale enhanced monocular depth estimation method
A depth estimation, multi-scale technology, applied in the computer vision field of deep learning, which can solve the problems of low accuracy of monocular depth estimation, low accuracy of depth map, and easy loss of intermediate layer feature information.
- Summary
- Abstract
- Description
- Claims
- Application Information
AI Technical Summary
Problems solved by technology
Method used
Image
Examples
Embodiment
[0067] The monocular depth estimation framework mentioned in this example is configured with two NVDIATitian Xp GPU hardware. The operating system used in this experiment is Windows, the deep learning framework is PyTorch, and the batch size is set to 4.
[0068] The data used in this embodiment is the NYU DepthV2 data set, which consists of 1449 pairs of RGB images and their corresponding images with depth information. In this embodiment, the officially divided training set and test set are used. Among them, 249 scenes are used as training set and 215 scenes are used as test set.
[0069] In addition, in order to improve the training speed of the model, the feature extraction part of the network framework (ABMN) proposed in this embodiment uses ImageNet[pre-trained parameters to initialize the front-end network, and uses the SGD optimizer to set the learning rate to is 0.0001, the momentum momentum is set to 0.9, and the weight decay weight_decay is set to 0.0005.
[0070] ...
PUM
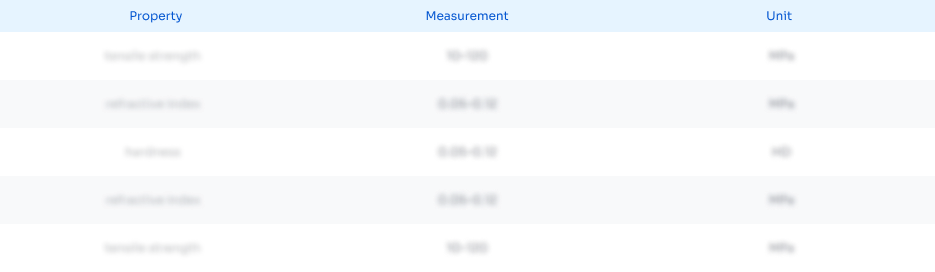
Abstract
Description
Claims
Application Information
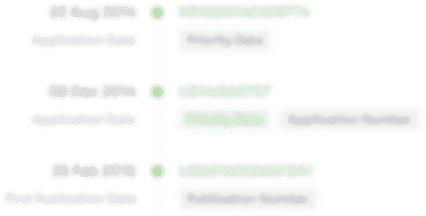
- R&D
- Intellectual Property
- Life Sciences
- Materials
- Tech Scout
- Unparalleled Data Quality
- Higher Quality Content
- 60% Fewer Hallucinations
Browse by: Latest US Patents, China's latest patents, Technical Efficacy Thesaurus, Application Domain, Technology Topic, Popular Technical Reports.
© 2025 PatSnap. All rights reserved.Legal|Privacy policy|Modern Slavery Act Transparency Statement|Sitemap|About US| Contact US: help@patsnap.com