Electric power data stream transmission time reasoning method based on graph neural network
A technology of power data and neural network, which is applied in the field of power data stream transmission time reasoning based on graph neural network, can solve problems such as long running time and inability to accurately reason about data stream transmission time, and achieve improved operating efficiency, good practical value, The effect of accurate reasoning
- Summary
- Abstract
- Description
- Claims
- Application Information
AI Technical Summary
Problems solved by technology
Method used
Image
Examples
Embodiment Construction
[0032] The following will clearly and completely describe the technical solutions in the embodiments of the present invention with reference to the accompanying drawings in the embodiments of the present invention. Obviously, the described embodiments are only some, not all, embodiments of the present invention. Based on the embodiments of the present invention, all other embodiments obtained by persons of ordinary skill in the art without making creative efforts belong to the protection scope of the present invention.
[0033] A graph neural network-based power data flow transmission time reasoning method, the steps are as follows:
[0034] Step 1. Establish the GNN model: establish the topology structure of the GNN model according to the network structure and routing information of the power data center, and map the network information and data flow information of the power data center to the characteristic values of the attribute graph in the GNN model.
[0035] E...
PUM
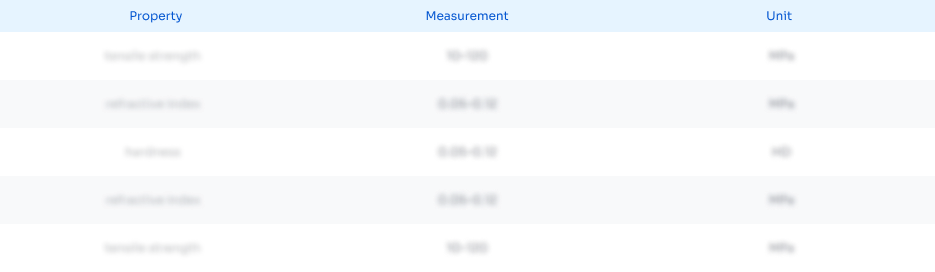
Abstract
Description
Claims
Application Information
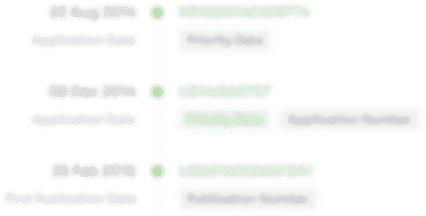
- R&D Engineer
- R&D Manager
- IP Professional
- Industry Leading Data Capabilities
- Powerful AI technology
- Patent DNA Extraction
Browse by: Latest US Patents, China's latest patents, Technical Efficacy Thesaurus, Application Domain, Technology Topic, Popular Technical Reports.
© 2024 PatSnap. All rights reserved.Legal|Privacy policy|Modern Slavery Act Transparency Statement|Sitemap|About US| Contact US: help@patsnap.com