Intelligent image annotation method
A technology of intelligent images and image files, applied in image enhancement, image analysis, image data processing, etc., can solve the problems of low data labeling efficiency, achieve the effects of shortening labeling time, improving labeling work, and improving efficiency
- Summary
- Abstract
- Description
- Claims
- Application Information
AI Technical Summary
Problems solved by technology
Method used
Image
Examples
Embodiment 1
[0032] Such as figure 1 As shown, an intelligent image labeling method includes the following steps:
[0033] Step 1: Obtain a database of image files to be marked, and select an image file as an OK sample;
[0034] Step 2: judge whether the match with the OK sample is successful;
[0035] Step 3: Manually compare the similarity between the matched file and the OK sample file;
[0036] Step 4: If the threshold value is appropriate and no adjustment is required, continue the previous operation; if the threshold value is not appropriate, it needs to be adjusted before repeating the previous operation, and then operate according to the similarity.
[0037] Step 1 includes the following sub-steps:
[0038] The first step: scramble the unlabeled data set, and then perform random extraction to extract a certain number of image data files;
[0039] The second step: mark the relevant defects on the extracted image data files;
[0040] The third step: classify the marked image dat...
Embodiment 2
[0051] Such as figure 1 As shown, an intelligent image labeling method includes the following steps:
[0052] Step 1: Obtain a database of image files to be marked, and select an image file as an OK sample;
[0053] Step 2: judge whether the match with the OK sample is successful;
[0054] Step 3: Manually compare the similarity between the matched file and the OK sample file;
[0055] Step 4: If the threshold value is appropriate and no adjustment is required, continue the previous operation; if the threshold value is not appropriate, it needs to be adjusted before repeating the previous operation, and then operate according to the similarity.
[0056] Step 1 includes the following sub-steps:
[0057] Step 1: Scramble the unlabeled data set, and then perform random extraction to extract a certain number of image data files; for example, if a certain amount of image data is extracted, then the remaining data sets can be set as samples to be extracted pond;
[0058] The se...
Embodiment 3
[0080] Such as figure 1 As shown, an intelligent image labeling method includes the following steps:
[0081] Step 1: Obtain a database of image files to be marked, and select an image file as an OK sample;
[0082] Step 2: judge whether the match with the OK sample is successful;
[0083] Step 3: Manually compare the similarity between the matched file and the OK sample file;
[0084] Step 4: If the threshold value is appropriate and no adjustment is required, continue the previous operation; if the threshold value is not appropriate, it needs to be adjusted before repeating the previous operation, and then operate according to the similarity.
[0085] In step 2, judge whether the match with the OK sample is successful. The specific operation is that you only need to run the script tool_retrieve.py, and follow the samples in the sample folder in one step, and automatically cut to the target folder.
[0086] In step 2, match the image data to be labeled with the sample data...
PUM
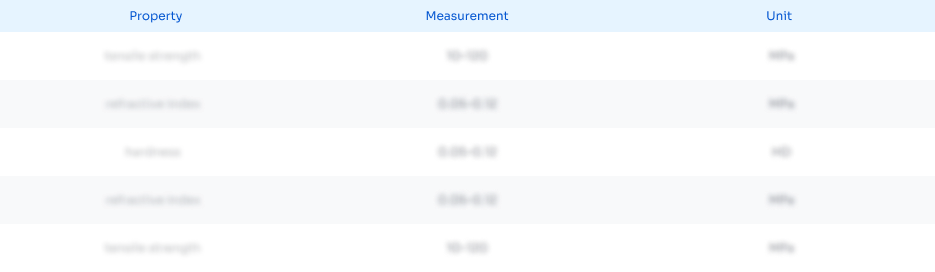
Abstract
Description
Claims
Application Information
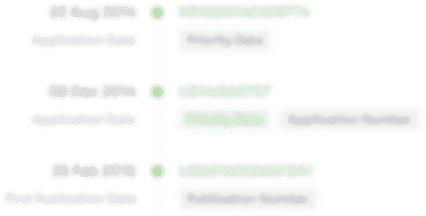
- R&D
- Intellectual Property
- Life Sciences
- Materials
- Tech Scout
- Unparalleled Data Quality
- Higher Quality Content
- 60% Fewer Hallucinations
Browse by: Latest US Patents, China's latest patents, Technical Efficacy Thesaurus, Application Domain, Technology Topic, Popular Technical Reports.
© 2025 PatSnap. All rights reserved.Legal|Privacy policy|Modern Slavery Act Transparency Statement|Sitemap|About US| Contact US: help@patsnap.com