Extraction method of radio and television news event elements based on deep learning
A radio and television, deep learning technology, applied in neural learning methods, electrical digital data processing, text database browsing/visualization, etc., can solve problems such as laborious and time-consuming, achieve good normalization discrimination, and improve the effect of extraction efficiency.
- Summary
- Abstract
- Description
- Claims
- Application Information
AI Technical Summary
Problems solved by technology
Method used
Image
Examples
Embodiment 1
[0054] Such as Figure 1~3 As shown, the method for extracting elements of radio and television news events based on deep learning includes steps:
[0055] S1, mark the abstract and element information of the radio and television news data to be analyzed, and construct the abstract data set and element data set;
[0056] S2, using the pre-trained model to construct a summary extraction model and an element extraction model, and using the abstract data set and the element data set constructed in step S1 to train the abstract extraction model and the element extraction model;
[0057] S3, using the abstract extraction model and element extraction model trained in step S2 to construct a two-stage radio and television news element automatic extraction model, using the model to predict the input radio and television news, and obtain a structured element extraction result.
Embodiment 2
[0059] On the basis of Embodiment 1, in step S1, constructing the feature data set is carried out on the basis of the abstract data set, including the following steps:
[0060] S11. Establish the core element lexicon of news events of N types of target types, use synonyms to expand the core element words, and then revise the expanded core element lexicon of news events, recall the news event data, and locate the core of the news event summary at the same time sentence; then the core element words are classified as the normalized expression of the core element; wherein, N is a positive integer;
[0061] S12, filter out the core sentences in the marked abstract according to the core elements of news events; sample and set the core sentences, summarize the characteristics of all other elements in the core sentences and their role category information in the news, and build elements for the follow-up The labeling system provides relevant configuration information and constraint in...
Embodiment 3
[0064] On the basis of embodiment 1, the summary extraction model in step S2 is recorded as the BertSum model, and the BertSum model is based on the Bert model, which adds a summary extraction layer based on Transformer on top of Bert to obtain sentence information as a summary.
[0065] The series sequence of the element extraction model in step S2 includes: text vectorization layer, core element extraction layer, other element extraction layer and core element normalized expression layer.
[0066] The text vectorization layer adopts the Bert layer after abstract extraction training fine-tune.
[0067] Considering that multiple news events may be described in a news core sentence, there are multiple core element words, and there may be nesting between these core element words (here, one core element word is inside another core element word, is its substring), so, in this embodiment, the construction process of the core element extraction layer includes the following steps:
...
PUM
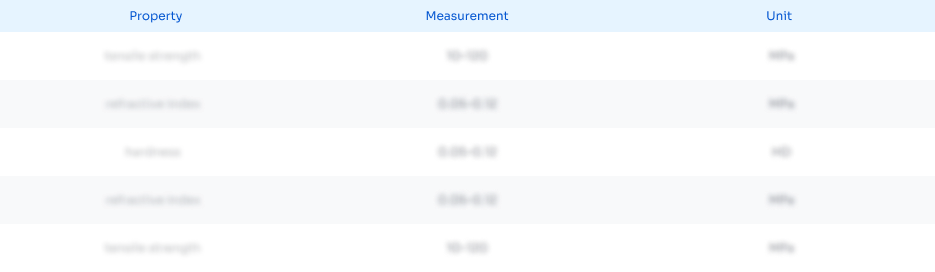
Abstract
Description
Claims
Application Information
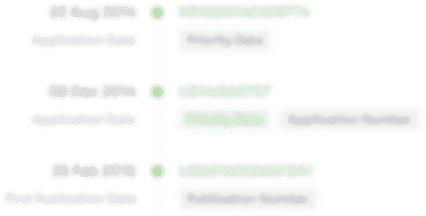
- R&D Engineer
- R&D Manager
- IP Professional
- Industry Leading Data Capabilities
- Powerful AI technology
- Patent DNA Extraction
Browse by: Latest US Patents, China's latest patents, Technical Efficacy Thesaurus, Application Domain, Technology Topic, Popular Technical Reports.
© 2024 PatSnap. All rights reserved.Legal|Privacy policy|Modern Slavery Act Transparency Statement|Sitemap|About US| Contact US: help@patsnap.com