An Active Crowdsourcing Image Learning Method Based on Semi-Supervised Variational Autoencoders
A technology of self-encoder and learning method, applied in the field of active crowdsourcing image learning, to achieve the effect of improving the effect and efficiency
- Summary
- Abstract
- Description
- Claims
- Application Information
AI Technical Summary
Problems solved by technology
Method used
Image
Examples
Embodiment Construction
[0046] Embodiments of the invention are described in detail below, examples of which are illustrated in the accompanying drawings. The embodiments described below by referring to the figures are exemplary only for explaining the present invention and should not be construed as limiting the present invention.
[0047] Such as figure 1 As shown, the specific process of the crowdsourcing image learning method based on semi-supervised variational autoencoder and active learning is shown, including the following steps:
[0048] Step 1: Construct an image crowdsourcing dataset.
[0049] After obtaining the image, randomly select t scaled images, denote them as , and then through crowdsourcing platforms, such as Amazon Mechanical Turk and Crowdflower, etc., it is distributed to the annotators on the network for pre-annotation, and the annotated . The rest of the unmarked part is denoted as .
[0050] Step 2: Build a semi-supervised crowdsourcing learning network model, fi...
PUM
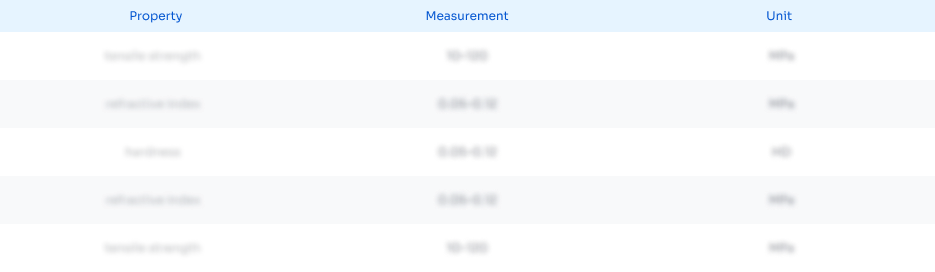
Abstract
Description
Claims
Application Information
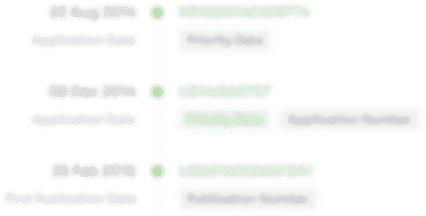
- R&D
- Intellectual Property
- Life Sciences
- Materials
- Tech Scout
- Unparalleled Data Quality
- Higher Quality Content
- 60% Fewer Hallucinations
Browse by: Latest US Patents, China's latest patents, Technical Efficacy Thesaurus, Application Domain, Technology Topic, Popular Technical Reports.
© 2025 PatSnap. All rights reserved.Legal|Privacy policy|Modern Slavery Act Transparency Statement|Sitemap|About US| Contact US: help@patsnap.com