Motion Boundary-Guided Optical Flow Filtering Method Based on Collaborative Deep Neural Networks
A deep neural network and motion boundary technology, applied in biological neural network models, neural architecture, image analysis, etc., can solve problems such as new errors and inaccurate modeling, improve efficiency and accuracy, and avoid introducing new errors Effect
- Summary
- Abstract
- Description
- Claims
- Application Information
AI Technical Summary
Problems solved by technology
Method used
Image
Examples
Embodiment Construction
[0024] The following will clearly and completely describe the technical solutions in the embodiments of the present invention with reference to the accompanying drawings in the embodiments of the present invention. Obviously, the described embodiments are only some, not all, embodiments of the present invention. Based on the embodiments of the present invention, all other embodiments obtained by persons of ordinary skill in the art without making creative efforts belong to the protection scope of the present invention.
[0025] Such as figure 1 As shown, the optical flow filtering method based on the motion boundary guidance of the collaborative deep neural network provided in this embodiment includes the following steps:
[0026] Step 1: Construct the optical flow filtering data set guided by the motion boundary, each sample in the data set includes the initial optical flow estimation result, motion boundary and optical flow true value. In the specific implementation process...
PUM
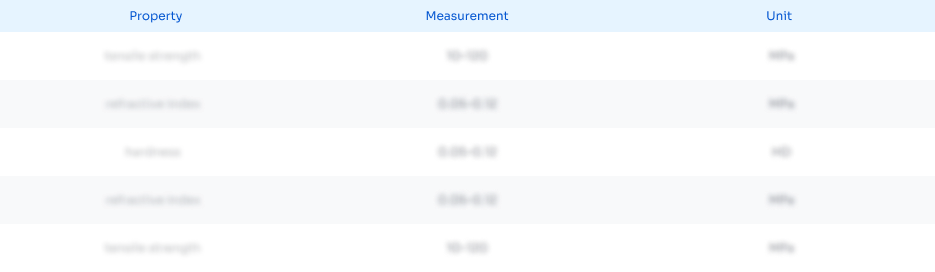
Abstract
Description
Claims
Application Information
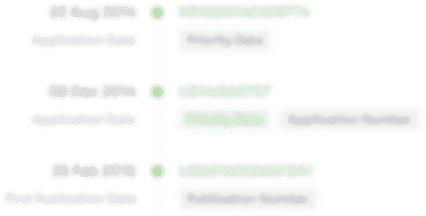
- R&D Engineer
- R&D Manager
- IP Professional
- Industry Leading Data Capabilities
- Powerful AI technology
- Patent DNA Extraction
Browse by: Latest US Patents, China's latest patents, Technical Efficacy Thesaurus, Application Domain, Technology Topic, Popular Technical Reports.
© 2024 PatSnap. All rights reserved.Legal|Privacy policy|Modern Slavery Act Transparency Statement|Sitemap|About US| Contact US: help@patsnap.com