Language model fine tuning method for low-resource adhesive language text classification
A language text and language model technology, applied in text database clustering/classification, semantic analysis, neural learning methods, etc., can solve high-uncertainty writing form vocabulary redundancy, difficulty in text classification, spelling and coding uncertainty sexual issues
- Summary
- Abstract
- Description
- Claims
- Application Information
AI Technical Summary
Problems solved by technology
Method used
Image
Examples
Embodiment 1
[0050] The step S1 uses the XLM-R model to model the language model. The XLM-R model uses the same shared vocabulary, randomly extracts sentences from the monolingual corpus for connection, learns BPE splitting, and processes language through byte pair encoding BPE , this method greatly improves the alignment of embedding spaces across languages that share the same alphabet or anchor tokens such as numbers or proper nouns.
[0051] The step S1 randomly extracting sentences is carried out according to multinomial distribution with probability, and its multinomial distribution is {q i} i =1,2,3,...n, specifically:
[0052]
[0053] in, And α=0.3.
[0054] This distributed sampling approach increases the number of tokens associated with low-resource languages and mitigates bias toward high-resource languages. In particular, words in low-resource languages can be prevented from being split at the character level.
Embodiment 2
[0056] The steps of fine-tuning the cross-lingual model in step S2 are:
[0057] S21: Using a suffix-based semi-supervised morpheme tokenizer, for candidate words, the semi-supervised morpheme tokenizer uses an iterative search algorithm to generate all word segmentation results by matching stem sets and suffix sets;
[0058] S22: When morphemes merge into words, the phonemes on the boundary change their surface morphology according to the rules of phonetics and writing, and the morphemes will coordinate with each other and appeal to each other's pronunciation;
[0059] S23: When the pronunciation is accurately represented, the phonetic harmony can be clearly observed in the text, and in the low-resource sticky text classification task, an independent statistical model is used to select the best result from the n best results ;
[0060] S24: Collect necessary terms by extracting word stems to form a fine-tuning data set with less noise, and then use the XLM-R model to fine-tu...
Embodiment 3
[0062] The concrete method of described step S3 discrimination fine-tuning is:
[0063] Different layers of the neural network can capture different levels of syntactic and semantic information. The lower layers of the XLM-R model may contain more general information. Use the classification learning rate to fine-tune the captured information, and divide the parameter θ into {θ 1 ,...,θ L}, where θL contains the parameters of the L-th layer, and the parameters are updated as follows:
[0064]
[0065] where η l Represents the learning rate of the L-th layer, t represents the update step, and the basic learning rate is set to η L , then η k-1 =ξ·η k , where ξ is the attenuation factor, and is less than or equal to 1; when ξ<1, the learning speed of the lower layer is slower than that of the upper layer; when ξ=1, all layers have the same learning rate, which is equivalent to regular stochastic gradient descent (SGD).
PUM
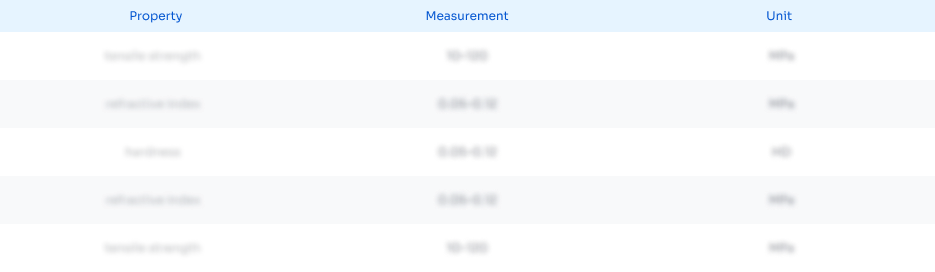
Abstract
Description
Claims
Application Information
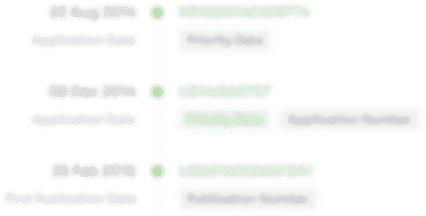
- R&D Engineer
- R&D Manager
- IP Professional
- Industry Leading Data Capabilities
- Powerful AI technology
- Patent DNA Extraction
Browse by: Latest US Patents, China's latest patents, Technical Efficacy Thesaurus, Application Domain, Technology Topic.
© 2024 PatSnap. All rights reserved.Legal|Privacy policy|Modern Slavery Act Transparency Statement|Sitemap