Adversarial attack and defense method and system based on prediction correction and stochastic step size optimization
A predictive correction and adversarial technology, applied in machine learning, computing models, computing, etc., can solve the loss of adversarial samples, cannot accurately evaluate the effectiveness of machine learning model robustness anti-defense methods, and cannot guarantee optimal disturbance range and other issues to achieve the effect of improving robustness and high attack success rate
- Summary
- Abstract
- Description
- Claims
- Application Information
AI Technical Summary
Problems solved by technology
Method used
Image
Examples
Embodiment
[0037] Such as figure 1 As shown, this embodiment conducts adversarial attacks and defensive attacks based on prediction correction and random step size optimization strategies, mainly involving the following technologies: 1) For adversarial attacks based on prediction correction and random step size optimization, the adversarial samples generated by existing methods As a predicted sample, the current disturbance is corrected using the gradient of the loss function relative to the predicted sample. At the same time, a random step is introduced in the process of generating adversarial samples, and the loss value of the sample obtained by the fixed step and random step is compared, and the sample with a larger loss value is selected as the adversarial sample. 2) Defense based on prediction correction and random step size optimization, using the adversarial samples generated by the adversarial attack method based on prediction correction and random step size optimization to condu...
PUM
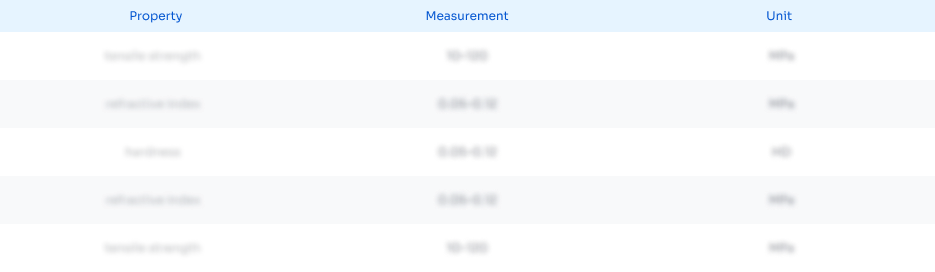
Abstract
Description
Claims
Application Information
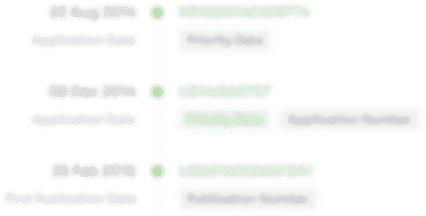
- R&D Engineer
- R&D Manager
- IP Professional
- Industry Leading Data Capabilities
- Powerful AI technology
- Patent DNA Extraction
Browse by: Latest US Patents, China's latest patents, Technical Efficacy Thesaurus, Application Domain, Technology Topic, Popular Technical Reports.
© 2024 PatSnap. All rights reserved.Legal|Privacy policy|Modern Slavery Act Transparency Statement|Sitemap|About US| Contact US: help@patsnap.com