MEMS sensor defect type identification method and system
An identification method and identification system technology, applied in the field of MEMS sensor surface defect detection, can solve problems such as insufficient training set, uncontrollable error rate, and product function impact
- Summary
- Abstract
- Description
- Claims
- Application Information
AI Technical Summary
Problems solved by technology
Method used
Image
Examples
Embodiment Construction
[0022] The present invention uses an improved confrontational neural network combined with a convolutional neural network as a method for MEMS sensor defect detection. The advantage of this method over traditional methods is that it can use the confrontational neural network to generate more data sets to improve the accuracy of the network. . The traditional adversarial generative neural network has only one set of discriminators and generators, while the improved adversarial generative neural network of the present invention uses multiple sets of discriminators and generators, which can directly generate labeled data without manually making labeled data. Directly put into the convolutional neural network for calculation to realize the automation of the detection process, which has important application value. The specific steps of the method for identifying the type of MEMS sensor defect will be introduced in detail below.
[0023] Step 1, acquire an image of the MEMS sensor...
PUM
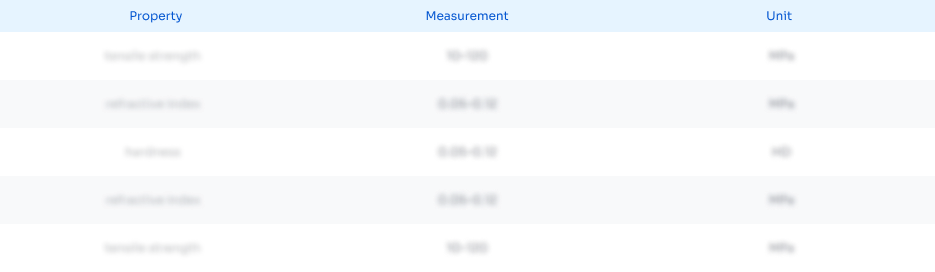
Abstract
Description
Claims
Application Information
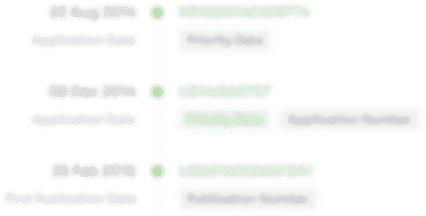
- R&D Engineer
- R&D Manager
- IP Professional
- Industry Leading Data Capabilities
- Powerful AI technology
- Patent DNA Extraction
Browse by: Latest US Patents, China's latest patents, Technical Efficacy Thesaurus, Application Domain, Technology Topic, Popular Technical Reports.
© 2024 PatSnap. All rights reserved.Legal|Privacy policy|Modern Slavery Act Transparency Statement|Sitemap|About US| Contact US: help@patsnap.com