Power battery prediction method based on big data transfer learning
A technology of power battery and transfer learning, which is applied in neural learning methods, biological neural network models, design optimization/simulation, etc., can solve problems such as increasing the difficulty of model development, less data accumulation for new products, and more product types, so as to improve the model Development efficiency, speed up model development, and reduce the difficulty of model development
- Summary
- Abstract
- Description
- Claims
- Application Information
AI Technical Summary
Problems solved by technology
Method used
Image
Examples
Embodiment 1
[0033] Embodiment 1: A power battery prediction method based on big data transfer learning, the method is: based on the big data of the power battery in advance, establish and train a transfer learning pre-training model with several reserved features for predicting the power battery ; When it is necessary to predict a new type of power battery, use part of the time series data of the new type of power battery to fine-tune the training migration learning pre-training model, and apply part of the features in the part of the time series data of the new type of power battery to the reserved features. Obtaining a new prediction model suitable for the new type of power battery; when predicting the power battery to be predicted belonging to the new type of power battery, using the new prediction model to predict the power battery to be predicted and obtaining a prediction result. In short, the power battery prediction method based on big data transfer learning in this application inc...
PUM
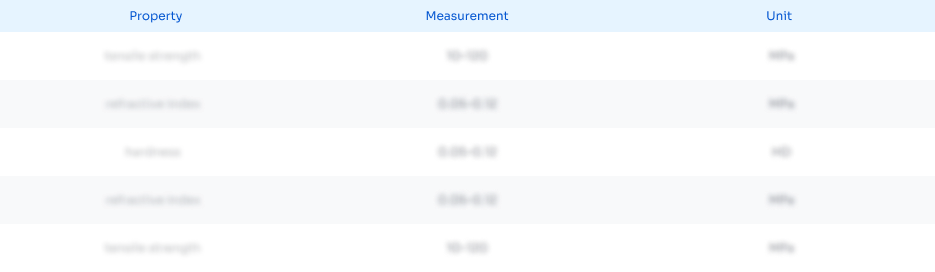
Abstract
Description
Claims
Application Information
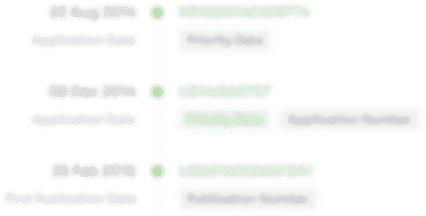
- R&D Engineer
- R&D Manager
- IP Professional
- Industry Leading Data Capabilities
- Powerful AI technology
- Patent DNA Extraction
Browse by: Latest US Patents, China's latest patents, Technical Efficacy Thesaurus, Application Domain, Technology Topic, Popular Technical Reports.
© 2024 PatSnap. All rights reserved.Legal|Privacy policy|Modern Slavery Act Transparency Statement|Sitemap|About US| Contact US: help@patsnap.com