Depth model for arrhythmia classification, and method and device utilizing model
A deep model and arrhythmia technology, applied in neural learning methods, biological neural network models, applications, etc., can solve the problems of few types of arrhythmia monitoring, huge model training costs, and low universality of ECG, etc., to achieve convenient deployment Effect
- Summary
- Abstract
- Description
- Claims
- Application Information
AI Technical Summary
Problems solved by technology
Method used
Image
Examples
Embodiment Construction
[0036] Hereinafter, the present application will be described in detail in conjunction with the accompanying drawings.
[0037] In view of the characteristics of the arrhythmia detection task, this application divides the heart beat classification process into two stages: representation learning and sequence learning. The overall training strategy and technical route are as follows: figure 2 As shown, it can be summarized into the following six steps.
[0038] 1) Analyze the ECG signal, and use the R wave detection algorithm to identify and mark the R peak, and then segment the original ECG signal: according to the sampling rate of the ECG signal collection device, set a fixed-size time-delay window to The R peak is the center, and the signal is truncated into a time series of equal length, which is convenient for feeding into the convolutional neural network structure. Preferably, the window size is set as a hyperparameter to obtain the optimal solution. Preferably, the ECG ...
PUM
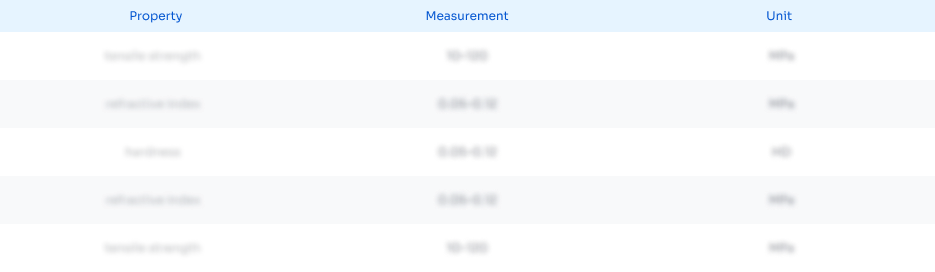
Abstract
Description
Claims
Application Information
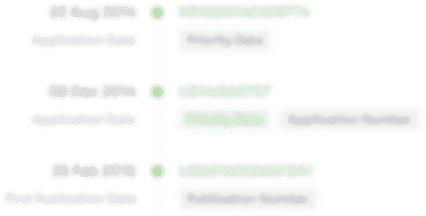
- R&D Engineer
- R&D Manager
- IP Professional
- Industry Leading Data Capabilities
- Powerful AI technology
- Patent DNA Extraction
Browse by: Latest US Patents, China's latest patents, Technical Efficacy Thesaurus, Application Domain, Technology Topic, Popular Technical Reports.
© 2024 PatSnap. All rights reserved.Legal|Privacy policy|Modern Slavery Act Transparency Statement|Sitemap|About US| Contact US: help@patsnap.com