Disordered grabbing multi-objective optimization method and system based on deep reinforcement learning
A technology of multi-objective optimization and reinforcement learning, applied in the field of multi-objective optimization of disordered grasping based on deep reinforcement learning, to achieve the effect of optimal selection
- Summary
- Abstract
- Description
- Claims
- Application Information
AI Technical Summary
Problems solved by technology
Method used
Image
Examples
Example Embodiment
[0060] Example 1
[0061] like figure 1 As shown, this Example 1 provides a unordered multi-objective optimization method based on deep strengthening learning, through two parallel independent Q networks, and handles the same scene at the same time, and the robot arms respectively Take the point to perform the grab, and return to the execution path, capture the power consumption and other parameters. Differentiate between the Q networks on the execution path, grab power consumption, etc., and generate corresponding reward values. Q Network accepts internal and external double reward function feedback, solving the reward value function of single Q network can only be discrete data, will execute the path, grab power consumption, etc., add continuous data to the reward value, so further optimization Select the selection of the point.
[0062] Specifically, the unordered multi-objective optimization method based on deep reinforced learning includes:
[0063] S110: Constructing a virtu...
Example Embodiment
[0101] Example 2
[0102] See figure 2 This embodiment provides a unordered multi-objective optimization system based on deep strengthening learning, the system comprising: virtual scene configuration module, task establishment module, virtual shooting module, output module, execution module, calculation module, feedback Modules and predictive model generation modules.
[0103] The virtual scene construction module is adapted to build a virtual scene of a mechanical arm.
[0104] The task establishment module is suitable for establishing two parallel independent depth enhanced learning network processing disorderly arrested multi-objective tasks. Specifically, the task establishment module is used to perform the following steps:
[0105] S121: Establish two parallel independent depth enhancement learning networks, which are the first network and the second network, where the network structure of the first network and the second network are the same;
[0106] S122: The network stru...
Example Embodiment
[0135] Example 3
[0136] This embodiment provides a computer readable storage medium that stores at least one instruction in the computer readable storage medium, and the instructions are executed by the processor to implement depth reinforced learning based on the embodiments provided by Example 1. Grab the multi-objective optimization method.
[0137] Distribution Multi-Objective Optimization Method Based on Deep Strengthening Learning By two parallel independent Q networks, the machine arm performs grabbing points for the respective grab points of the two networks, and returns the execution path. Grab the power consumption and other parameters. Differentiate between the Q networks on the execution path, grab power consumption, etc., and generate corresponding reward values. Q Network accepts internal and external double reward function feedback, solving the reward value function of single Q network can only be discrete data, will execute the path, grab power consumption, etc.,...
PUM
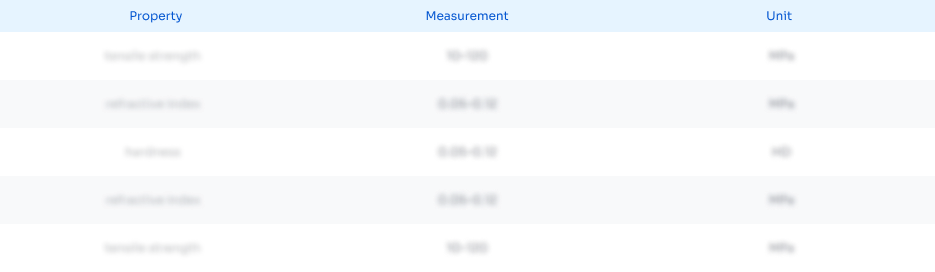
Abstract
Description
Claims
Application Information
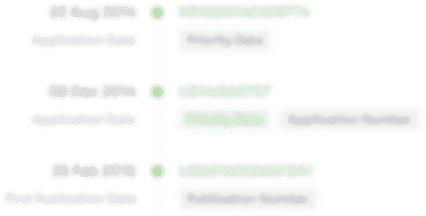
- R&D Engineer
- R&D Manager
- IP Professional
- Industry Leading Data Capabilities
- Powerful AI technology
- Patent DNA Extraction
Browse by: Latest US Patents, China's latest patents, Technical Efficacy Thesaurus, Application Domain, Technology Topic.
© 2024 PatSnap. All rights reserved.Legal|Privacy policy|Modern Slavery Act Transparency Statement|Sitemap