Driver fatigue driving real-time detection method and system based on deep learning
A driver fatigue, real-time detection technology, applied in the field of computer vision and deep learning, can solve the problems of inability to meet the real-time requirements of fatigue driving detection, few data sets for fatigue driving detection, and many deep neural network parameters, etc., to improve reliability. performance and practicability, avoiding repeated extraction, and reducing the amount of computation
- Summary
- Abstract
- Description
- Claims
- Application Information
AI Technical Summary
Problems solved by technology
Method used
Image
Examples
Embodiment 1
[0026] Such as figure 1 As shown, a kind of driver's fatigue driving real-time detection method based on deep learning that the embodiment of the present invention provides, comprises the following steps:
[0027] Step S1: Collect the real-time image of the driver's cab, use the three-level cascaded neural network to detect the position of the face, and use the face position of the previous frame in the subsequent frame to use the third level of the three-level cascaded neural network. Face tracking to obtain face images;
[0028] Step S2: Normalize the size, mean and variance of the face image to obtain the face input feature map, input the face key point detection deep neural network, and obtain the face key points;
[0029] Step S3: Select part of the key points of the face and match them with the key points of the 3D standard face to obtain the 3D posture of the head, and identify the behavior of nodding and not looking straight ahead according to the multi-frame results;...
Embodiment 2
[0072] Such as Figure 6 As shown, the embodiment of the present invention provides a real-time detection system for driver fatigue driving based on deep learning, including the following modules:
[0073] Obtaining face image module 61, used to collect real-time images of the driver's cab, using a three-level cascaded neural network to detect the position of the face, and in subsequent frames, using the face position of the previous frame, using a three-level cascaded neural network The third stage in the process carries out face tracking to obtain a face image;
[0074] Obtaining the human face key point module 62 is used to normalize the size, mean and variance of the human face image to obtain the input feature map of the human face, input the human face key point detection depth neural network, and obtain the human face key point;
[0075] Identifying nodding and not looking straight ahead module 63, used to select some key points of the face and match them with 3D stand...
PUM
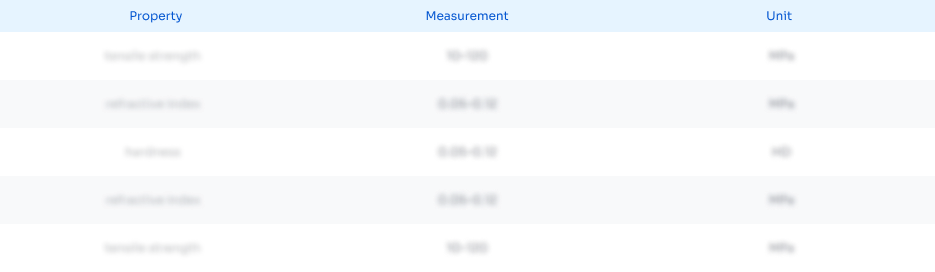
Abstract
Description
Claims
Application Information
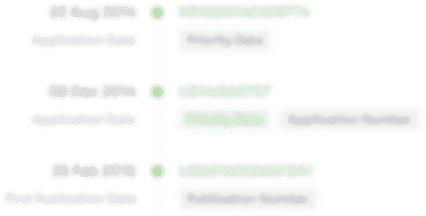
- R&D
- Intellectual Property
- Life Sciences
- Materials
- Tech Scout
- Unparalleled Data Quality
- Higher Quality Content
- 60% Fewer Hallucinations
Browse by: Latest US Patents, China's latest patents, Technical Efficacy Thesaurus, Application Domain, Technology Topic, Popular Technical Reports.
© 2025 PatSnap. All rights reserved.Legal|Privacy policy|Modern Slavery Act Transparency Statement|Sitemap|About US| Contact US: help@patsnap.com