Robust multi-tag feature selection method considering feature label dependency
A feature selection method and feature labeling technology, applied in complex mathematical operations, instruments, character and pattern recognition, etc., can solve the problems of losing key information and wrong labels
- Summary
- Abstract
- Description
- Claims
- Application Information
AI Technical Summary
Problems solved by technology
Method used
Image
Examples
Embodiment Construction
[0054] See figure 1 , Specific implementation steps are as follows:
[0055] Step 1: Enter the feature matrix Label matrix Among them, N represents the number of training samples, D represents the characteristic dimension of the training sample, and C represents the label dimension of the training sample;
[0056] The regular mean coefficient α, β, gamma ∈ {0.001, 0.01, 0.1, 1, 10, 100, 1000}, maximum iterative number m> 0, convergence threshold ∈> 0, potential semantic matrix dimensions R> 0 and weighting factor Δ> 0;
[0057] Step 2: Initializing the least squares diagonal matrix And sparse regular diagonal matrix Unit to angular matrix;
[0058] Step 3: Latent Semantic random initialization matrix of the matrix in the range [0, 1] And corresponding coefficient matrices
[0059] Step 4: Set the initial value of the target function θ 0 And an initial value of the number of iterations t = 0;
[0060] Step 5: Judging whether the convergence condition is met Skip to step 9 if...
PUM
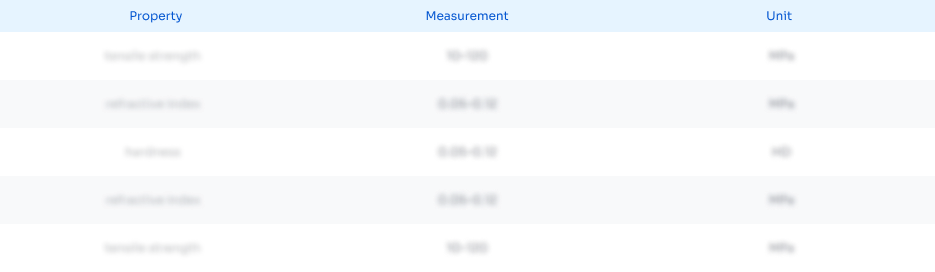
Abstract
Description
Claims
Application Information
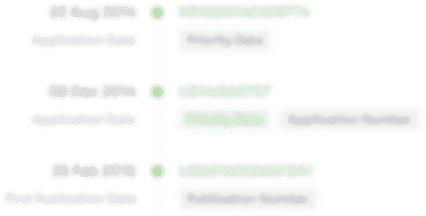
- R&D Engineer
- R&D Manager
- IP Professional
- Industry Leading Data Capabilities
- Powerful AI technology
- Patent DNA Extraction
Browse by: Latest US Patents, China's latest patents, Technical Efficacy Thesaurus, Application Domain, Technology Topic, Popular Technical Reports.
© 2024 PatSnap. All rights reserved.Legal|Privacy policy|Modern Slavery Act Transparency Statement|Sitemap|About US| Contact US: help@patsnap.com