Stock trend prediction method of fused graph convolutional network
A convolutional network and trend forecasting technology, applied in forecasting, neural learning methods, biological neural network models, etc., can solve the problems of traditional models such as real-time performance and unsatisfactory prediction accuracy
- Summary
- Abstract
- Description
- Claims
- Application Information
AI Technical Summary
Problems solved by technology
Method used
Image
Examples
Embodiment Construction
[0039] The technical solutions in the embodiments of the present invention will be clearly and completely described below in conjunction with the accompanying drawings in the embodiments of the present invention. Obviously, the described embodiments are only some of the embodiments of the present invention, not all of them. Based on this The embodiments in the invention, and all other embodiments obtained by persons of ordinary skill in the art without creative efforts, all belong to the scope of protection of the present invention.
[0040] Such as Figure 1-Figure 3 As shown, an embodiment of a stock trend prediction method fused with a graph convolutional network described in this embodiment includes the following steps.
[0041] Step 1. Use knowledge in the financial field to construct various relationship graphs between stocks, such as equity graphs, industry graphs, and topical graphs.
[0042] Equity Chart G X = (V,E X , A X ) is used to encode the equity impact, a...
PUM
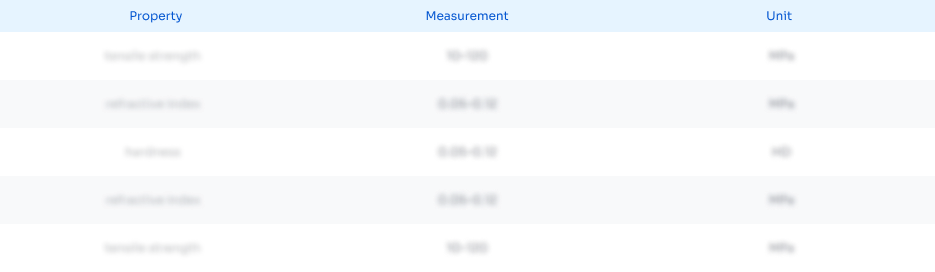
Abstract
Description
Claims
Application Information
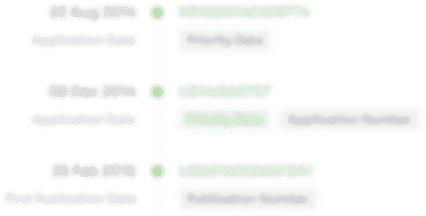
- R&D
- Intellectual Property
- Life Sciences
- Materials
- Tech Scout
- Unparalleled Data Quality
- Higher Quality Content
- 60% Fewer Hallucinations
Browse by: Latest US Patents, China's latest patents, Technical Efficacy Thesaurus, Application Domain, Technology Topic, Popular Technical Reports.
© 2025 PatSnap. All rights reserved.Legal|Privacy policy|Modern Slavery Act Transparency Statement|Sitemap|About US| Contact US: help@patsnap.com