Cellular network-oriented improved reinforcement learning network coverage optimization method
A technology of reinforcement learning and network coverage, applied in the field of communication networks, it can solve the problems of multiple preparations, large amount of calculation, inability to use network resources efficiently, etc., and achieve the effect of avoiding impact and fast convergence speed.
- Summary
- Abstract
- Description
- Claims
- Application Information
AI Technical Summary
Problems solved by technology
Method used
Image
Examples
Embodiment Construction
[0089] The improved reinforcement learning network coverage optimization method for cellular networks described in this embodiment, the flow chart is as follows figure 1 shown, including:
[0090] (1) from figure 2 In a heterogeneous wireless network environment, terminal drive test data and base station static data are collected to form data samples. After preprocessing, the data samples are divided into normal coverage samples, weak coverage samples, and excessive coverage samples. According to the engineering parameters of each data sample The weight and the number of coverage samples of each class are processed for the three coverage samples to obtain a balanced data set;
[0091] Preprocessing in the step 1 includes:
[0092] (101) Data splicing: the static data on the base station side includes the base station number and the cell number, the terminal drive test data includes the cell number accessed by the sample, and the static data on the base station side with the...
PUM
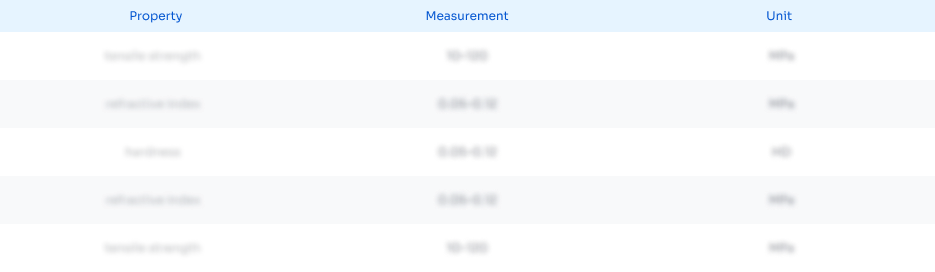
Abstract
Description
Claims
Application Information
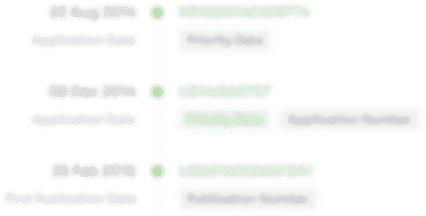
- R&D
- Intellectual Property
- Life Sciences
- Materials
- Tech Scout
- Unparalleled Data Quality
- Higher Quality Content
- 60% Fewer Hallucinations
Browse by: Latest US Patents, China's latest patents, Technical Efficacy Thesaurus, Application Domain, Technology Topic, Popular Technical Reports.
© 2025 PatSnap. All rights reserved.Legal|Privacy policy|Modern Slavery Act Transparency Statement|Sitemap|About US| Contact US: help@patsnap.com