Adversarial sample generation method and system based on image brightness random transformation
A technology against samples and image brightness, which is applied in the directions of graphic image conversion, image data processing, neural learning methods, etc., can solve the problems of low attack success rate and performance differences, achieve good application prospects, eliminate overfitting, and improve reliability migratory effect
- Summary
- Abstract
- Description
- Claims
- Application Information
AI Technical Summary
Problems solved by technology
Method used
Image
Examples
Embodiment Construction
[0024] In order to make the purpose, technical solutions and advantages of the present invention more clear and understandable, the present invention will be described in further detail below in conjunction with the accompanying drawings and technical solutions.
[0025] The deep neural network is vulnerable to adversarial samples. This kind of adversarial sample is to add noise that is almost invisible to human vision on the original input image, so that the deep neural network can be misclassified, which poses a threat to the deep neural network. Therefore, before the deployment of deep neural networks, adversarial attacks can be used as an important method to evaluate the robustness of the model. However, in the case of black boxes, the attack success rate of adversarial samples needs to be improved, that is, the transferability of adversarial samples needs to be improved. An embodiment of the present invention provides a method for generating an adversarial example based o...
PUM
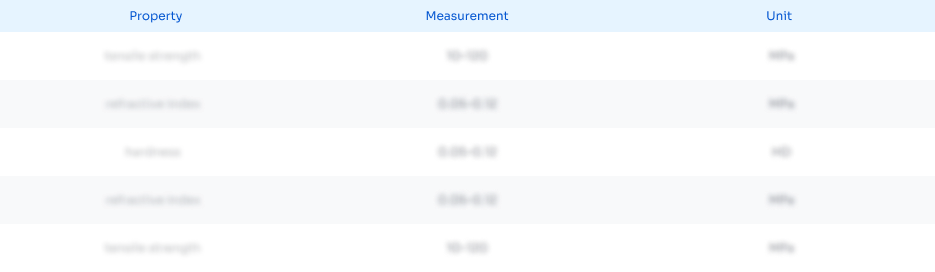
Abstract
Description
Claims
Application Information
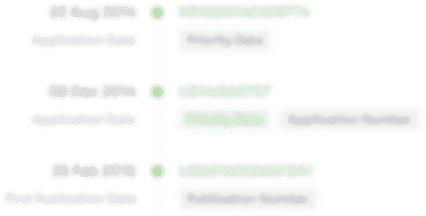
- R&D
- Intellectual Property
- Life Sciences
- Materials
- Tech Scout
- Unparalleled Data Quality
- Higher Quality Content
- 60% Fewer Hallucinations
Browse by: Latest US Patents, China's latest patents, Technical Efficacy Thesaurus, Application Domain, Technology Topic, Popular Technical Reports.
© 2025 PatSnap. All rights reserved.Legal|Privacy policy|Modern Slavery Act Transparency Statement|Sitemap|About US| Contact US: help@patsnap.com