Adversarial sample defense method and device based on data disturbance
A technology against samples and data, applied in the direction of neural learning methods, combustion engines, internal combustion piston engines, etc., can solve the problems of long training period, inflexible defense methods, and difficulty in meeting the actual needs of autonomous driving scenarios, so as to improve the recognition accuracy Effect
- Summary
- Abstract
- Description
- Claims
- Application Information
AI Technical Summary
Problems solved by technology
Method used
Image
Examples
Embodiment Construction
[0051] The technical content of the present invention will be described in detail below in conjunction with the accompanying drawings and specific embodiments.
[0052] figure 1 A schematic diagram of the application of the adversarial example defense method provided by the present invention in the automatic driving scene. In the autonomous driving scenario, the neural network model H(·) is implanted into the autonomous vehicle as the recognition neural network, and the data disturbance is the interference factor that affects the recognition image of the autonomous vehicle. The image here is the image information acquired by the self-driving vehicle during driving, such as street signs, road surfaces, roadblocks, and pedestrians.
[0053] Such as figure 2 As shown, the adversarial sample defense method provided by the embodiment of the present invention at least includes the following steps:
[0054] 101. Add data perturbation to input samples to form defense samples;
[...
PUM
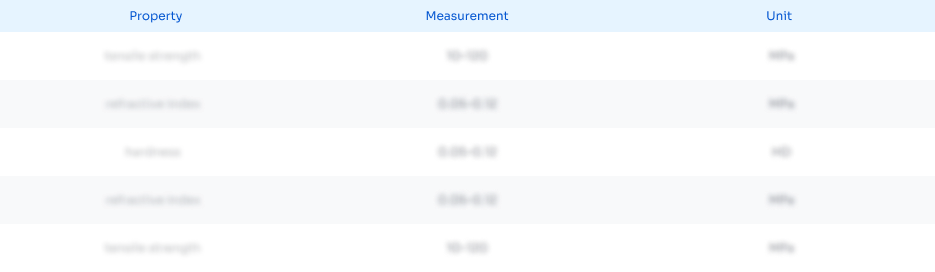
Abstract
Description
Claims
Application Information
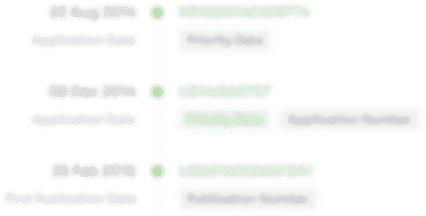
- R&D
- Intellectual Property
- Life Sciences
- Materials
- Tech Scout
- Unparalleled Data Quality
- Higher Quality Content
- 60% Fewer Hallucinations
Browse by: Latest US Patents, China's latest patents, Technical Efficacy Thesaurus, Application Domain, Technology Topic, Popular Technical Reports.
© 2025 PatSnap. All rights reserved.Legal|Privacy policy|Modern Slavery Act Transparency Statement|Sitemap|About US| Contact US: help@patsnap.com