Analog signal identification method based on complex neural network and attention mechanism
An analog signal and neural network technology, applied in the field of analog signal recognition, can solve the problem that the meta-learning method has not been studied, and achieve the effect of excellent innovation and good classification effect.
- Summary
- Abstract
- Description
- Claims
- Application Information
AI Technical Summary
Problems solved by technology
Method used
Image
Examples
Embodiment 1
[0111] Embodiment 1 experiment and verification
[0112] In order to make the purpose and effect of the present invention clearer, take the signal prediction experiment of the meta-learning model CAMEL based on the complex neural network and the attention mechanism of the present invention as an example, and use the analog signal data set RADIOML 2016.04C to analyze the integrated model of the present invention Describe in detail.
[0113] S1.1: The dimension of the original input data is 2×128, and its classification labels are 11 modulation modes: 8PSK, AM-DSB, AM-SSB, BPSK, CPFSK, GFSK, PAM4, QAM16, QAM64, QPSK, WBFM. The input signal signal-to-noise ratio SNR ranges from -20dB to 20dB, and this experiment only selects signal samples with SNR≥0;
[0114] S1.2: Divide the data set into P (Prediction) set and O (Other) set, select 5 types of samples to form P set, and the other 6 types of samples to form O set. Select 95% of the samples in the P set to form the test set, an...
Embodiment 2
[0139] Example 2 actual scene
[0140] Use the network model after the optimized parameters trained in the last step of Example 1 above, and perform iterative training to continuously optimize the CAMEL network model at the same time, use the test data set to test its final performance, and apply it in the actual prediction work.
[0141] For example, at the base station, the modem (Modem) module of equipment such as mobile phones is used to collect IQ signals (for analog signal data, divided into training sets and test sets), with reference to embodiment 1 step S1.1, the dimension of the original input data is 2 × 128, so that the data dimension is 2×128, wherein the first dimension is 2, representing the real part and the imaginary part of the complex data. Screen the signal data with a suitable signal-to-noise ratio (SNR), the signal data with SNR greater than or equal to 0, and prepare for input prediction.
[0142] Divide the data into training set and test set, input th...
PUM
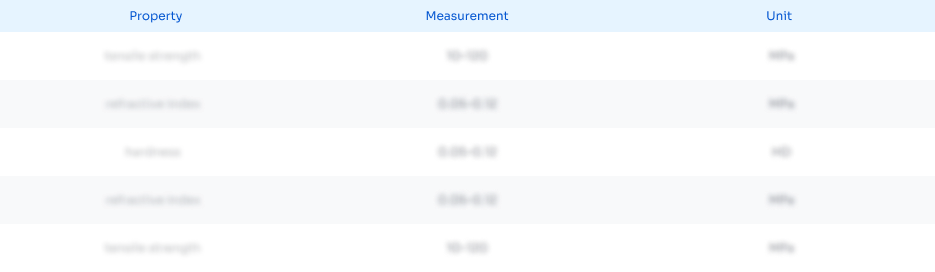
Abstract
Description
Claims
Application Information
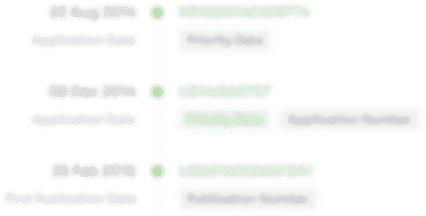
- R&D Engineer
- R&D Manager
- IP Professional
- Industry Leading Data Capabilities
- Powerful AI technology
- Patent DNA Extraction
Browse by: Latest US Patents, China's latest patents, Technical Efficacy Thesaurus, Application Domain, Technology Topic.
© 2024 PatSnap. All rights reserved.Legal|Privacy policy|Modern Slavery Act Transparency Statement|Sitemap