Classification detection method and device based on lightweight classification model
A classification model and classification detection technology, applied in neural learning methods, biological neural network models, character and pattern recognition, etc., can solve the problems of low product image detection efficiency and low production efficiency, and achieve small initialization width and light classification model Quantity, good detection effect
- Summary
- Abstract
- Description
- Claims
- Application Information
AI Technical Summary
Problems solved by technology
Method used
Image
Examples
Embodiment 1
[0066] Such as figure 1 As shown, Embodiment 1 provides a classification detection method based on a lightweight classification model, including the following steps:
[0067] S101. Acquire a training sample image, and determine a category of the training sample image.
[0068] In step S101, the training sample images are in different industrial inspection fields. In order to ensure the accuracy of the product category classification of the lightweight classification model built according to the actual detection situation in each industrial inspection field, a sufficient number training set images. Input enough training set images, that is, training sample images, into the constructed lightweight classification model, so that the lightweight classification model can learn enough training set images; that is, the characteristics of the training sample images, and extract them according to the detection Each training set image, that is, the characteristics of the training sampl...
Embodiment 2
[0113] Corresponding to the foregoing embodiment 1 of a classification detection method based on a lightweight classification model, the present application also provides a classification detection device based on a lightweight classification model. The classification detection device includes:
[0114] Training module and classification detection module;
[0115] Wherein, the training module is used for:
[0116] Obtain a training sample image, and determine the category of the training sample image;
[0117] Classifying and marking the training sample images according to the categories to obtain sample marked images;
[0118] Inputting the sample marked image into a lightweight classification model for training to obtain a trained lightweight classification model;
[0119] The classification detection module is used for:
[0120] Inputting the sample image to be evaluated into the trained lightweight classification model, judging and outputting the category to which the ...
PUM
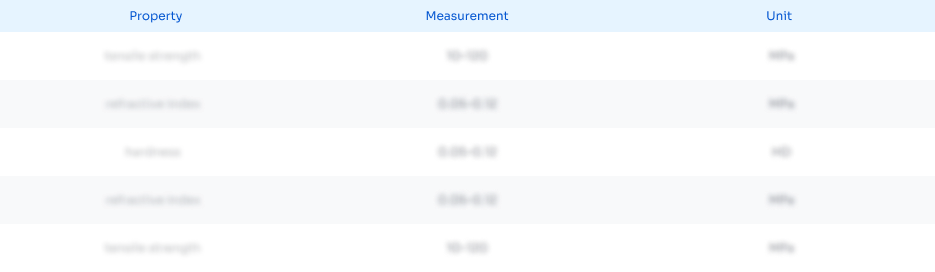
Abstract
Description
Claims
Application Information
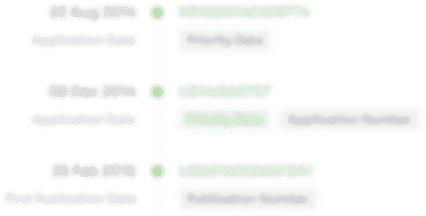
- R&D Engineer
- R&D Manager
- IP Professional
- Industry Leading Data Capabilities
- Powerful AI technology
- Patent DNA Extraction
Browse by: Latest US Patents, China's latest patents, Technical Efficacy Thesaurus, Application Domain, Technology Topic, Popular Technical Reports.
© 2024 PatSnap. All rights reserved.Legal|Privacy policy|Modern Slavery Act Transparency Statement|Sitemap|About US| Contact US: help@patsnap.com