Compressed image recognition method based on deep learning
A technology of compressing images and constructing methods, which is applied in the field of compressed image recognition based on deep learning, can solve the problems of exacerbating compressed image feature drift, loss of details, and limiting recognition performance, so as to improve classification performance, enhance features, and improve recognition accuracy. Effect
- Summary
- Abstract
- Description
- Claims
- Application Information
AI Technical Summary
Problems solved by technology
Method used
Image
Examples
Embodiment 1
[0031] This embodiment is combined with a lossless picture data set Div2k containing a large number of natural scenes. Among them, the data is saved in a PNG format, a total of 2000 non-destructive original pictures that do not compress as a raw training image. Technical solutions to the present invention (main processes such as figure 1 The shown is specifically described.
[0032] First, design features enhancement modules, such as figure 2 As shown, including the following steps:
[0033] Step 1: Select the desired pre-training classification model and the corresponding feature location.
[0034] In particular, the pre-training classification model can use common classic architecture, such as VGG16 networks, and SQUEEZENET networks. When using the VGG16 network, the CONV2-2 layer of the network is selected as the characteristic enhanced position; when using the SQUEEZENET network, select the first layer of the network as the position of the feature enhancement.
[0035] The VGG...
PUM
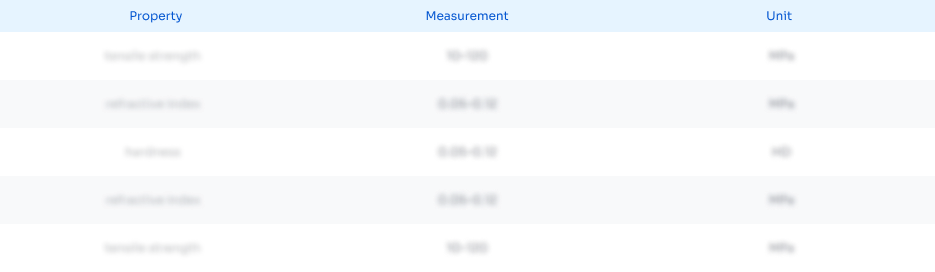
Abstract
Description
Claims
Application Information
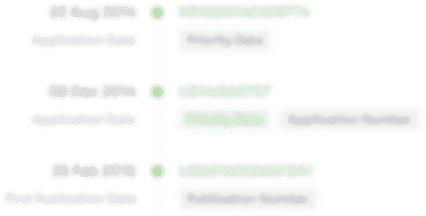
- R&D Engineer
- R&D Manager
- IP Professional
- Industry Leading Data Capabilities
- Powerful AI technology
- Patent DNA Extraction
Browse by: Latest US Patents, China's latest patents, Technical Efficacy Thesaurus, Application Domain, Technology Topic, Popular Technical Reports.
© 2024 PatSnap. All rights reserved.Legal|Privacy policy|Modern Slavery Act Transparency Statement|Sitemap|About US| Contact US: help@patsnap.com