Grouping model construction method based on causal inference and medical data processing method
A construction method and model technology, which is applied in the construction of grouping models based on causal inference and in the field of medical data processing, can solve problems such as distribution deviation, low accuracy, and unreasonableness, and achieve the effect of eliminating selection bias and high accuracy
- Summary
- Abstract
- Description
- Claims
- Application Information
AI Technical Summary
Problems solved by technology
Method used
Image
Examples
Embodiment 1
[0063] see figure 1 , shows a flow chart of the steps of the method for constructing a grouping model based on causal inference according to an embodiment of the present invention. It can be understood that the flowchart in this method embodiment is not used to limit the sequence of execution steps. The following is an exemplary description taking computer equipment as the execution subject, as follows:
[0064] Such as figure 1 As shown, the method for constructing a grouping model based on causal inference may include steps S100 to S106, wherein:
[0065] In step S100, a plurality of sample data of a plurality of sample patients is acquired, and the plurality of sample data of each sample patient includes a plurality of basic data, a plurality of patient history follow-up data and sample patient grouping result data.
[0066] In an exemplary embodiment, the plurality of sample patients may be a plurality of diabetic patients. The historical follow-up data of multiple dia...
Embodiment 2
[0093] read on Figure 4 , shows a schematic diagram of the program modules of the causal inference-based grouping model construction system of the present invention. In this embodiment, the grouping model construction system 40 based on causal inference may include or be divided into one or more program modules, and one or more program modules are stored in a storage medium and executed by one or more processors. Execute to complete the present invention, and realize the above-mentioned method for constructing a grouping model based on causal inference. The program module referred to in the embodiment of the present invention refers to a series of computer program instruction segments capable of completing specific functions, which is more suitable than the program itself to describe the execution process of the causal inference-based grouping model construction system 40 in the storage medium. The following description will specifically introduce the functions of each progr...
Embodiment 3
[0119] read on Image 6 , shows a schematic diagram of program modules of the medical data processing system of the present invention. In this embodiment, the medical data processing system 60 may include or be divided into one or more program modules, and one or more program modules are stored in a storage medium and executed by one or more processors to complete The present invention can also realize the above-mentioned medical data processing method. The program module referred to in the embodiment of the present invention refers to a series of computer program instruction segments capable of completing specific functions, which is more suitable for describing the execution process of the medical data processing system 60 in the storage medium than the program itself. The following description will specifically introduce the functions of each program module of the present embodiment:
[0120] The medical data processing system includes:
[0121] The second acquiring modu...
PUM
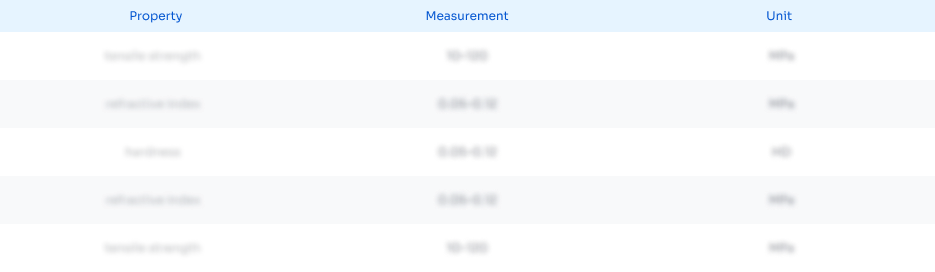
Abstract
Description
Claims
Application Information
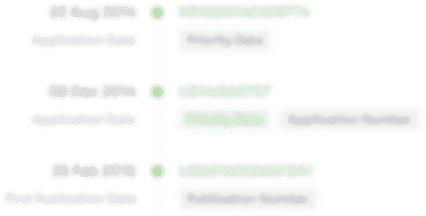
- R&D Engineer
- R&D Manager
- IP Professional
- Industry Leading Data Capabilities
- Powerful AI technology
- Patent DNA Extraction
Browse by: Latest US Patents, China's latest patents, Technical Efficacy Thesaurus, Application Domain, Technology Topic, Popular Technical Reports.
© 2024 PatSnap. All rights reserved.Legal|Privacy policy|Modern Slavery Act Transparency Statement|Sitemap|About US| Contact US: help@patsnap.com