Named entity recognition method based on multistage context feature extraction
A named entity recognition and feature extraction technology, applied in the direction of neural learning methods, instruments, biological neural network models, etc., can solve the problems of unable to extract global information, model degradation, etc., and achieve the effect of solving the problem of model degradation
- Summary
- Abstract
- Description
- Claims
- Application Information
AI Technical Summary
Problems solved by technology
Method used
Image
Examples
Embodiment Construction
[0066] Such as figure 1 As shown, bidirectional long short-term memory neural network (Bi-LSTM), as one of the effective networks for sequence labeling tasks, has been widely used in named entity recognition. However, due to the sequential nature of Bi-LSTM and the inability to recognize multiple sentences at the same time, it cannot obtain global information. To make up for the deficiency of Bi-LSTM in extracting global information, the present invention proposes a hierarchical context model embedded with sentence-level and document-level feature extraction. In the sentence-level feature extraction, considering the different contributions of each word to the sentence, the present invention uses a self-attention mechanism to extract sentence-level expressions. For document-level feature extraction, 3D convolutional neural network (CNN) can not only extract features within sentences, but also pay attention to the order relationship between sentences, so the present invention u...
PUM
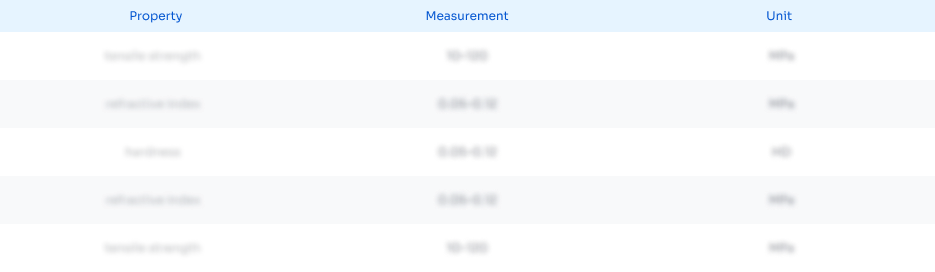
Abstract
Description
Claims
Application Information
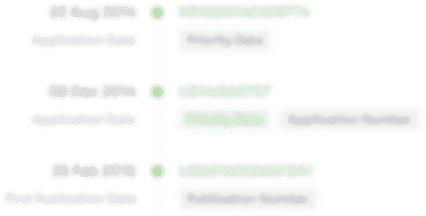
- R&D Engineer
- R&D Manager
- IP Professional
- Industry Leading Data Capabilities
- Powerful AI technology
- Patent DNA Extraction
Browse by: Latest US Patents, China's latest patents, Technical Efficacy Thesaurus, Application Domain, Technology Topic.
© 2024 PatSnap. All rights reserved.Legal|Privacy policy|Modern Slavery Act Transparency Statement|Sitemap