Resource scheduling method, resource scheduling system and equipment
A resource scheduling and resource technology, applied in the computer field, can solve the problems of reduced operating efficiency, resources cannot meet CRD resource requests, CRD cannot be scheduled, etc., and achieves the effect of high scheduling efficiency
- Summary
- Abstract
- Description
- Claims
- Application Information
AI Technical Summary
Problems solved by technology
Method used
Image
Examples
Embodiment 1
[0095] This embodiment is an example of the scheduler successfully scheduling CRDs and Pods. The embodiment shows the process of mixing CRDs and Pods on the Kubernetes scheduling platform. The deep learning job is defined as a CRD, and the workers who complete the deep learning job in parallel are assigned to the Pod. Bearer, which can realize the mixed scheduling of deep learning jobs and Pods and can run successfully.
[0096] Instance environment: a Kubernetes cluster equipped with Ubuntu 16.04 system, including two nodes with sufficient resources; the cluster has deployed a modified scheduler; deployed a custom deep learning job controller and splitter.
[0097] see Figure 7 , the specific operation steps are as follows:
[0098] Step S710: define a deep learning job file, and create the CRD object;
[0099] Step S720: define a single Pod file, and create the Pod object;
[0100] Step S730: After the deep learning job is successfully created, the CRD corresponding to t...
Embodiment 2
[0104] In this example, the scheduler successfully schedules two types of CRD objects. The example shows the process of mixing and scheduling different CRDs on the Kubernetes scheduling platform. The deep learning job is defined as a CRD, and the machine learning job is defined as a CRD, and the two types of CRD objects are executed. All Workers are carried by Pods, which can realize the mixed scheduling of deep learning jobs and machine learning jobs, and all of them can run successfully.
[0105] Instance environment: Kubernetes cluster equipped with Ubuntu 16.04 system, including two nodes with sufficient resources; the cluster has deployed a modified scheduler; deployed a custom deep learning job controller and splitter; deployed a custom machine Controller and splitter for learning jobs.
[0106] see Figure 8 , the specific operation steps are as follows:
[0107] Step S810: define the file of the deep learning job, and create the CRD object;
[0108] Step S820: defin...
Embodiment 3
[0115] In this embodiment, the scheduler schedules CRDs to run on the fewest nodes. The embodiment shows that when scheduling CRD objects on the Kubernetes scheduling platform, CRDs can be reasonably dismantled according to resource status, and deep learning jobs are defined as CRDs to complete deep learning. Workers that execute jobs in parallel are carried by Pods. When scheduling CRDs, the scheduler can automatically dismantle CRDs according to the current resource status, and schedule CRD Pods to run on extremely small nodes, reducing network overhead and ensuring the rationality of dismantling. .
[0116] Instance environment: Kubernetes cluster equipped with Ubuntu 16.04 system, including 3 nodes, the CPU and memory resources of the nodes are sufficient, node 1 has 8 idle GPUs, nodes 2 and 3 have 4 idle GPUs; the cluster has deployed the modified Scheduler; controller and splitter for deploying custom deep learning jobs.
[0117] see Figure 9 , the specific operation ...
PUM
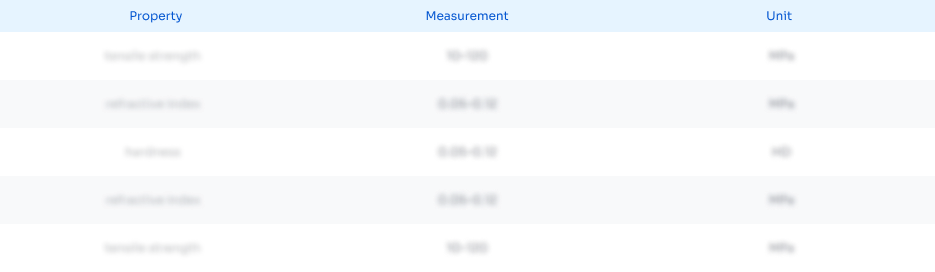
Abstract
Description
Claims
Application Information
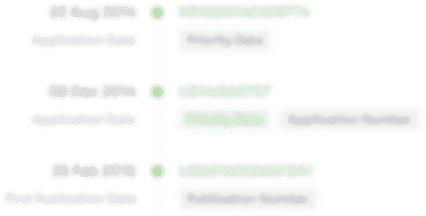
- R&D Engineer
- R&D Manager
- IP Professional
- Industry Leading Data Capabilities
- Powerful AI technology
- Patent DNA Extraction
Browse by: Latest US Patents, China's latest patents, Technical Efficacy Thesaurus, Application Domain, Technology Topic, Popular Technical Reports.
© 2024 PatSnap. All rights reserved.Legal|Privacy policy|Modern Slavery Act Transparency Statement|Sitemap|About US| Contact US: help@patsnap.com