LSTM energy consumption prediction method based on dual feature selection and particle swarm optimization
A technology of particle swarm optimization and feature selection, applied in prediction, neural learning methods, biological neural network models, etc., can solve problems such as unstable prediction performance, low energy consumption prediction accuracy, and unsatisfactory building energy consumption prediction, etc., to achieve The model algorithm has high efficiency, good model fitting effect, and stable prediction performance effect
- Summary
- Abstract
- Description
- Claims
- Application Information
AI Technical Summary
Problems solved by technology
Method used
Image
Examples
Embodiment
[0114] Now, the present invention will be described in detail by taking the power consumption prediction of a certain building as an example of the trial application of the present invention, which also has a guiding effect on the application of the present invention to other building energy consumption predictions.
[0115] In this implementation, the historical power consumption of a building is used as a time series to predict the power consumption of a short-term single-step 1h.
[0116] In this embodiment, the electricity consumption forecast of a certain building includes the following contents:
[0117] 1. Experimental data set and MI feature selection
[0118] The data set used in this example is the electricity consumption of a certain building from October 15, 2019 to June 4, 2019. The data set has a total of 20 features. A description of these features is shown in Table 1. The data in the fifth column is the pearsonr correlation coefficient value between the curre...
PUM
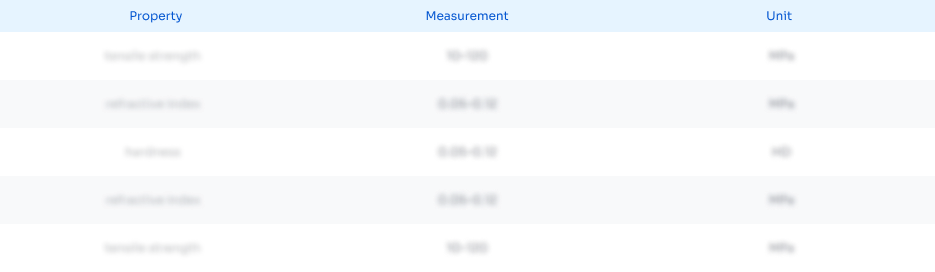
Abstract
Description
Claims
Application Information
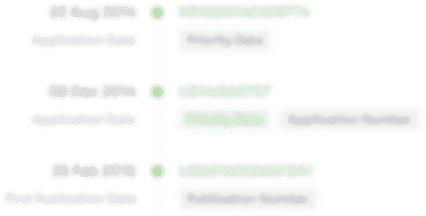
- R&D Engineer
- R&D Manager
- IP Professional
- Industry Leading Data Capabilities
- Powerful AI technology
- Patent DNA Extraction
Browse by: Latest US Patents, China's latest patents, Technical Efficacy Thesaurus, Application Domain, Technology Topic.
© 2024 PatSnap. All rights reserved.Legal|Privacy policy|Modern Slavery Act Transparency Statement|Sitemap