An Image Generation Method Based on Small-Sample Continuous Learning
An image generation and small sample technology, applied in the field of deep learning image processing, can solve the problems of difficulty in realizing small sample learning and continuous learning at the same time, and affect the performance of task sequence processing, so as to save training resources and improve computing efficiency.
- Summary
- Abstract
- Description
- Claims
- Application Information
AI Technical Summary
Problems solved by technology
Method used
Image
Examples
Embodiment Construction
[0043] The present invention provides an image generation method based on continuous learning of small samples, such as figure 1 As shown, the specific steps are:
[0044] S1: Data set construction, constructing pre-training data sets and continuous learning data sets:
[0045] pre-training stage, such as figure 2 As shown in the training phase 1 of the pre-training dataset It is a large-scale data set, and the continuous learning data set is a small sample data set. pre-training dataset The samples in the continuous learning data set are composed of real images and their semantic annotations. The amount of image data corresponding to each semantic in the pre-training data set is large. The continuous learning data set includes semantics that are not in the pre-training data set. Continuous learning The data set is divided into subtask dataset , each subtask dataset contained in Semantics that do not appear in .
[0046] S2: Construct the generator model in the...
PUM
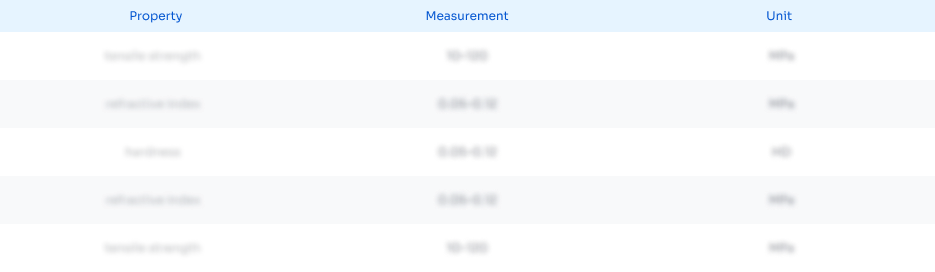
Abstract
Description
Claims
Application Information
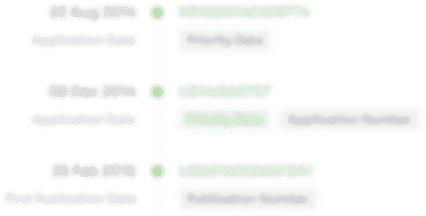
- R&D Engineer
- R&D Manager
- IP Professional
- Industry Leading Data Capabilities
- Powerful AI technology
- Patent DNA Extraction
Browse by: Latest US Patents, China's latest patents, Technical Efficacy Thesaurus, Application Domain, Technology Topic, Popular Technical Reports.
© 2024 PatSnap. All rights reserved.Legal|Privacy policy|Modern Slavery Act Transparency Statement|Sitemap|About US| Contact US: help@patsnap.com