Target detection method based on selectable expansion convolution kernel size
A target detection and convolution kernel technology, which is applied to instruments, character and pattern recognition, computer components, etc., can solve problems such as increasing computational complexity and difficulty in achieving real-time
- Summary
- Abstract
- Description
- Claims
- Application Information
AI Technical Summary
Problems solved by technology
Method used
Image
Examples
Embodiment Construction
[0013] The present invention will be further described below in conjunction with accompanying drawing:
[0014] The method of constructing an optional dilated convolution module is as follows:
[0015] A series of feature layers, pooling layers and activation layers are obtained through the Darknet-53 network, and P in Darknet-53 5 , P 4 The feature layers are sequentially introduced into SDCM, and sent to the corresponding feature layers in the upsampling stage for weighted fusion.
[0016] The network consists of a convolution function (conv), an activation function (leakyrelu) and an average pooling function (avgpool). Given an input F, F obtains an output F' after selecting an expansion coefficient formula, and F' is first obtained through a channel attention mechanism f C , and then the spatial attention mechanism gets the final output F".
[0017] F'=w×conv 1(F)+(1-w)×conv 2(F) (1)
[0018] In the formula, the convolution coefficient of the conv1 function is 1, the ...
PUM
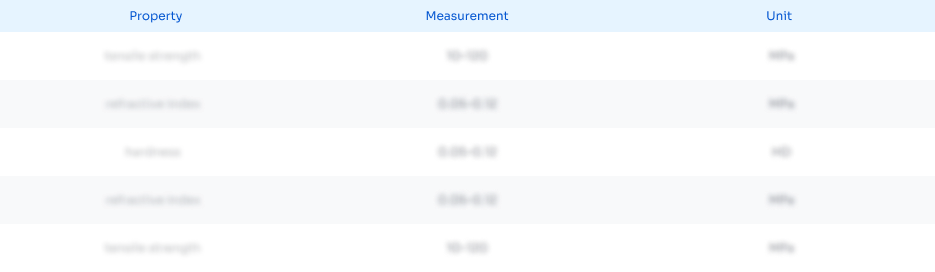
Abstract
Description
Claims
Application Information
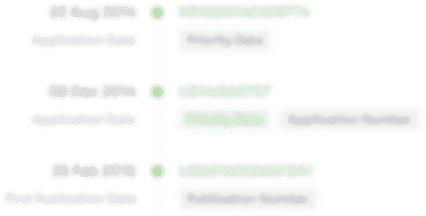
- R&D
- Intellectual Property
- Life Sciences
- Materials
- Tech Scout
- Unparalleled Data Quality
- Higher Quality Content
- 60% Fewer Hallucinations
Browse by: Latest US Patents, China's latest patents, Technical Efficacy Thesaurus, Application Domain, Technology Topic, Popular Technical Reports.
© 2025 PatSnap. All rights reserved.Legal|Privacy policy|Modern Slavery Act Transparency Statement|Sitemap|About US| Contact US: help@patsnap.com