Distribution line pin defect detection method based on improved ALI and Faster-RCNN
A distribution line and defect detection technology, applied in the direction of reasoning methods, neural learning methods, character and pattern recognition, etc., can solve the problem that hidden information cannot be well represented, pins are not easy to detect, model recognition, pin defect detection Problems such as low accuracy rate can achieve the effect of reducing training complexity, avoiding probability calculation problems, and enhancing local texture
- Summary
- Abstract
- Description
- Claims
- Application Information
AI Technical Summary
Problems solved by technology
Method used
Image
Examples
Embodiment Construction
[0040] Such as figure 1 As shown, based on the improved adversarial learning inference (ALI) and Faster-RCNN distribution line pin defect detection method, this method proposes an improved adversarial learning inference ALI model, and the original distribution line pin image data to be detected is used as a training sample , ALI is used as a training algorithm to train the model, and the pin detection image data is used to learn the ALI network model through the trained and improved adversarial inference to generate a reconstructed pin image dataset; finally, the trained Faster-RCNN model is used to detect whether the image exists Pin defects, the method comprising the steps of:
[0041] Step 1. Data acquisition:
[0042] The original image was collected by drone inspection. The size of the image is 5000×3500, and the pins account for less than 1% of the entire image. This dataset mainly collects the pin damage of power distribution lines. The state of the pin is divided int...
PUM
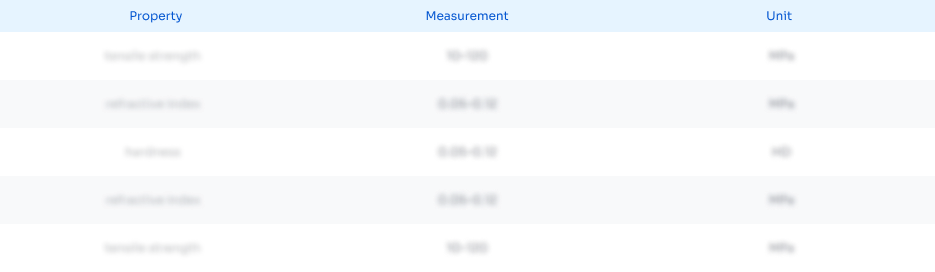
Abstract
Description
Claims
Application Information
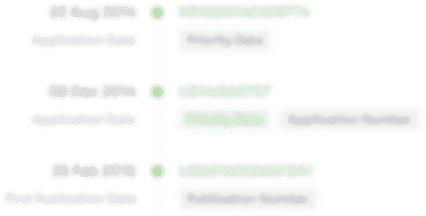
- R&D Engineer
- R&D Manager
- IP Professional
- Industry Leading Data Capabilities
- Powerful AI technology
- Patent DNA Extraction
Browse by: Latest US Patents, China's latest patents, Technical Efficacy Thesaurus, Application Domain, Technology Topic, Popular Technical Reports.
© 2024 PatSnap. All rights reserved.Legal|Privacy policy|Modern Slavery Act Transparency Statement|Sitemap|About US| Contact US: help@patsnap.com