Federal mutual learning model training method for non-independent identically distributed data
A distributed data and learning model technology, which is applied in the field of federated mutual learning model training for non-independent and identically distributed data, can solve the problems of high training performance, lack of communication costs, and reduce direct communication between clients and cloud servers to achieve shortened models the effect of time
- Summary
- Abstract
- Description
- Claims
- Application Information
AI Technical Summary
Problems solved by technology
Method used
Image
Examples
Embodiment 1
[0066] According to the method steps provided in this embodiment, a model training method for solving non-IID federated learning is introduced, taking the MNIST image classification task as an example. Each client is a data owner, at least one terminal participates in training, and intermediate clients participate. The sample data held by each data owner can be the same data set or different data sets. The server and client databases store the MNIST data set. After manual segmentation, the edge clients in each group only contain one or more disjoint data labels, satisfying the situation of non-independent and identical distribution. The server, intermediate client, and edge client use the LeNet-5 convolutional neural network (the input layer dimension is 28×28, the convolution layer includes six 5×5 convolution kernels, and the maximum pooling layer includes a 2 ×2 kernel, the convolution layer includes 16 5×5 convolution kernels, the maximum pooling layer includes 1 2×2 kern...
PUM
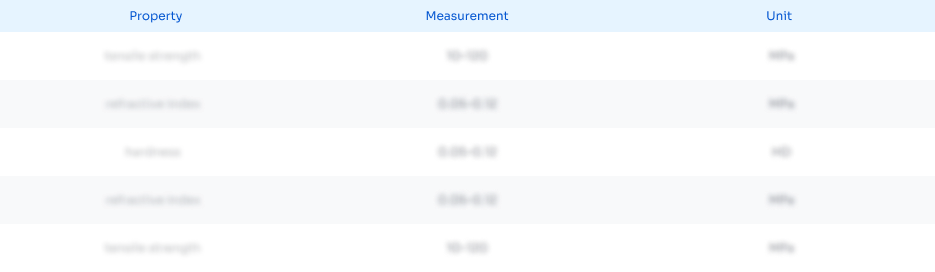
Abstract
Description
Claims
Application Information
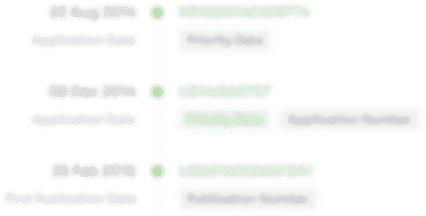
- R&D Engineer
- R&D Manager
- IP Professional
- Industry Leading Data Capabilities
- Powerful AI technology
- Patent DNA Extraction
Browse by: Latest US Patents, China's latest patents, Technical Efficacy Thesaurus, Application Domain, Technology Topic, Popular Technical Reports.
© 2024 PatSnap. All rights reserved.Legal|Privacy policy|Modern Slavery Act Transparency Statement|Sitemap|About US| Contact US: help@patsnap.com