YOLOv5 neural network vehicle detection method added with attention mechanism
A neural network and vehicle detection technology, applied in the field of image detection and recognition, can solve the problems of no allocation, affecting image recognition, errors, etc., and achieve the effect of improving the recognition accuracy, the speed of model convergence, and the effect of improving the effect.
- Summary
- Abstract
- Description
- Claims
- Application Information
AI Technical Summary
Problems solved by technology
Method used
Image
Examples
Embodiment 1
[0035] refer to Figure 1~6 , is an embodiment of the present invention, provides a kind of YOLOv5 neural network vehicle detection method that adds attention mechanism, comprises:
[0036] S1: Use the car image dataset and preprocess the dataset.
[0037] It should be noted that the UA-DETRAC public dataset is used, the image labeling tool Labelimg is used to label the image, and the COCO dataset format is saved as a txt format label file, and the dataset is divided into a training set according to a division ratio of 8:1. and validation set.
[0038] S2: Improve the YOLOv5 neural network and add an attention mechanism.
[0039] It should be noted that, if the channel attention module is added, the input feature map, that is, H×W×C, is passed through the global max pooling layer (global max pooling) and the global average based on H and W respectively. Pooling (global average pooling) to obtain two 1×1×C feature maps; send the feature maps to a two-layer neural network (ML...
Embodiment 2
[0058] This embodiment is another embodiment of the present invention. What this embodiment is different from the first embodiment is that it provides a verification test of the YOLOv5 neural network vehicle detection method that adds attention mechanism, which is the technology adopted in this method. The effect is verified and explained. In this embodiment, the traditional technical solution is used to conduct a comparative test with the method of the present invention, and the test results are compared by means of scientific demonstration to verify the real effect of the method.
[0059] In this example, the training CPU is Intel Core i5-6300HQ@2.30GHz, the GPU is GTX1080Ti, the deep learning framework used is Pytorch1.9.0, and the CUDA version is 11.2, and then follow the steps in Example 1 to input the image data into the unimproved YOLOv5 Train and test in the network, compare the test results of the two, and the results are shown in the following table:
[0060] Table 1...
PUM
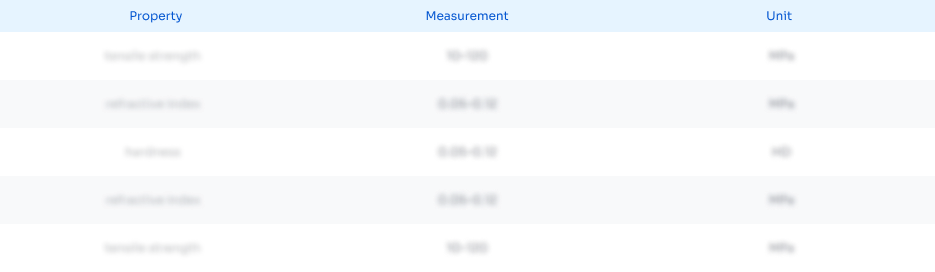
Abstract
Description
Claims
Application Information
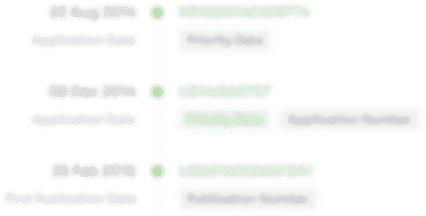
- Generate Ideas
- Intellectual Property
- Life Sciences
- Materials
- Tech Scout
- Unparalleled Data Quality
- Higher Quality Content
- 60% Fewer Hallucinations
Browse by: Latest US Patents, China's latest patents, Technical Efficacy Thesaurus, Application Domain, Technology Topic, Popular Technical Reports.
© 2025 PatSnap. All rights reserved.Legal|Privacy policy|Modern Slavery Act Transparency Statement|Sitemap|About US| Contact US: help@patsnap.com