Radiation dose distribution prediction method and device based on CT image
A CT image and radiation dose technology, applied in the field of radiation dose distribution prediction methods and devices, to achieve the effect of improving accuracy and maintaining topology
- Summary
- Abstract
- Description
- Claims
- Application Information
AI Technical Summary
Problems solved by technology
Method used
Image
Examples
Embodiment 1
[0068] Please refer to figure 1 , a radiation dose distribution prediction method based on CT images, comprising steps:
[0069] S1. Obtain a CT image to be predicted;
[0070] S2. Extract the first feature map and the second feature map of two different resolutions in the CT image to be predicted through the convolutional neural network, and the first initial differential field and the second feature map corresponding to the first feature map The second initial differential field corresponding to the feature map;
[0071] Among them, please refer to figure 2 , the convolutional neural network includes a first convolutional flow, a second convolutional flow and a third convolutional flow, the first convolutional flow is the convolutional neural network input to the CNN node at the branch, so The second convolution flow is the first CNN branch at the branch, and the second convolution flow is the second CNN branch at the branch; using a decoding branch structure similar to ...
Embodiment 2
[0089] The difference between this embodiment and Embodiment 1 is that a field regularization mechanism (Field Regularization) is also provided to supervise the loss of the training process;
[0090] specific:
[0091] Set the loss function: L=L Dice (Y 1 , Y label )+L Grad (φ FT )+L Grad (φ Bulk ), where L Dice is the dice loss function, Y 1 For the comparison sample, Y label is the predicted sample, that is, the predicted result, φ FT is the first differential field, φ Bulk is the second differential field, L Grad for:
[0092]
[0093] h and w are the parameters corresponding to the first feature map and the second feature map, that is, if calculating L Grad (φ FT ), bring in h and w corresponding to the first feature map, if calculate L Grad (φ Bulk ) is brought into h and w corresponding to the second feature map;
[0094] In an optional implementation manner, the loss function also includes a weighting parameter β;
[0095] Then L=L Dice (Y, Y label ...
Embodiment 3
[0097] Please refer to figure 2 , this embodiment provides a specific dose distribution prediction network framework structure;
[0098] The image input on the left is the CT image to be predicted as Y, the image input on the lower right is the prior image as P, and the image output on the upper right is the predicted value image as Y label ;
[0099] The first convolution flow includes five CNN nodes and four maximum pooling processes in turn; the bottleneck branch of the first convolution flow is the second convolution flow and the third convolution flow; the second convolution flow The second convolution flow is composed of a CNN node (upsampling block) and 1×1 convolution, and the third convolution flow is composed of three CNN nodes and 1×1 convolution; Matrix splicing is performed on corresponding CNN nodes in the first convolution flow to generate a two-dimensional field u, namely the first initial differential field and the second initial differential field;
[010...
PUM
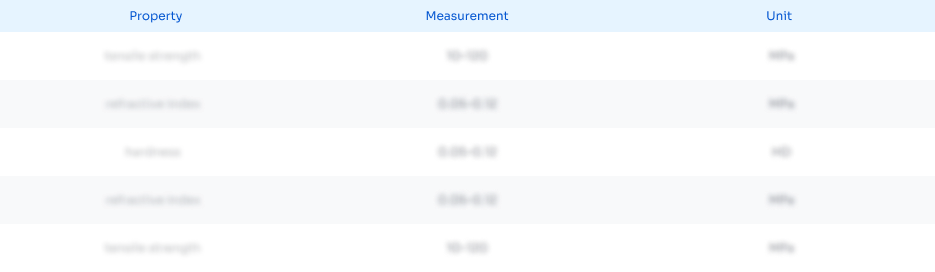
Abstract
Description
Claims
Application Information
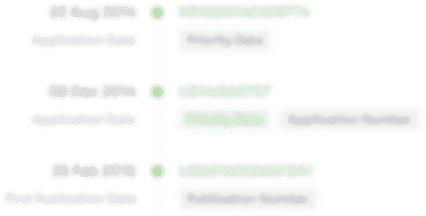
- R&D Engineer
- R&D Manager
- IP Professional
- Industry Leading Data Capabilities
- Powerful AI technology
- Patent DNA Extraction
Browse by: Latest US Patents, China's latest patents, Technical Efficacy Thesaurus, Application Domain, Technology Topic.
© 2024 PatSnap. All rights reserved.Legal|Privacy policy|Modern Slavery Act Transparency Statement|Sitemap