Target tracking method based on task distinguishing detection and re-identification joint network
A re-identification and task technology, applied in the field of computer vision, can solve problems such as not being able to adapt to different tasks, and achieve the effect of improving accuracy
- Summary
- Abstract
- Description
- Claims
- Application Information
AI Technical Summary
Problems solved by technology
Method used
Image
Examples
Embodiment Construction
[0028] As mentioned above, the present invention proposes a target tracking method based on task differentiation, detection and re-identification joint network. The specific implementation of the present invention will be described below with reference to the accompanying drawings.
[0029] (1) Overall process
[0030] The invention proposes a joint network of task differentiation, detection and recognition to realize video multi-target tracking. The overall framework diagram of the joint network of task differentiation, detection and re-identification is shown in the attached figure 1 As shown, it mainly includes three parts: (1) backbone network; (2) multi-feature fusion network; (3) multi-task branch. These three parts are also three steps of the method proposed by the present invention.
[0031] For the input current frame, first use the DLA backbone network (as attached figure 2 (shown) extracts the shared features used in the target detection task and target re-ident...
PUM
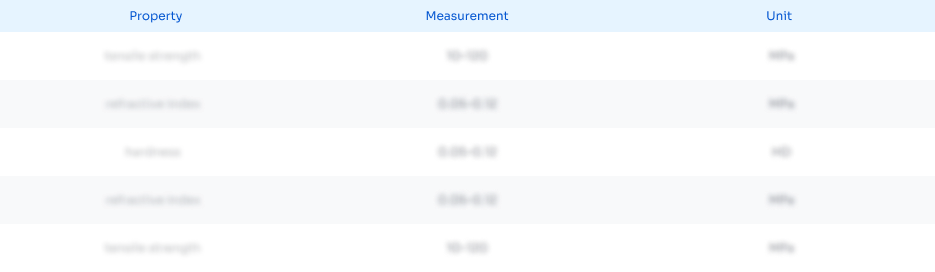
Abstract
Description
Claims
Application Information
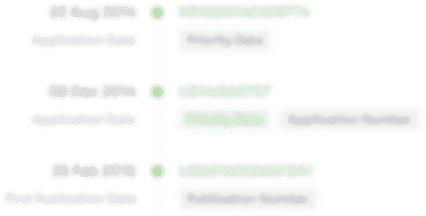
- Generate Ideas
- Intellectual Property
- Life Sciences
- Materials
- Tech Scout
- Unparalleled Data Quality
- Higher Quality Content
- 60% Fewer Hallucinations
Browse by: Latest US Patents, China's latest patents, Technical Efficacy Thesaurus, Application Domain, Technology Topic, Popular Technical Reports.
© 2025 PatSnap. All rights reserved.Legal|Privacy policy|Modern Slavery Act Transparency Statement|Sitemap|About US| Contact US: help@patsnap.com