Future trajectory prediction in multi-actor environment for autonomous machine applications
A kind of actor and corresponding technology, applied in special data processing applications, instruments, computer components, etc., can solve the problem of invalid real-time deployment of runtime
- Summary
- Abstract
- Description
- Claims
- Application Information
AI Technical Summary
Problems solved by technology
Method used
Image
Examples
Embodiment Construction
[0019] Systems and methods related to future trajectory prediction in a multi-agent environment (using one or more deep neural networks (DNNs)) for autonomous machine applications are disclosed. While the present disclosure may be described with respect to an example ego vehicle 600 (herein alternatively referred to as "vehicle 600" or "ego vehicle 600"), with respect to Figure 6A to Figure 6D Examples thereof are described, but this is not intended to be limiting. For example, the systems and methods described herein may be implemented by, but not limited to, non-autonomous vehicles, semi-autonomous vehicles (e.g., in one or more Adaptive Driver Assistance Systems (ADAS)), robots, warehouse vehicles, off-road vehicles, flying Watercraft, boats, shuttles, emergency response vehicles, motorcycles, e-bikes or mopeds, aircraft, construction vehicles, underwater boats, drones and / or other vehicle types. Additionally, while the present disclosure may be described with respect to ...
PUM
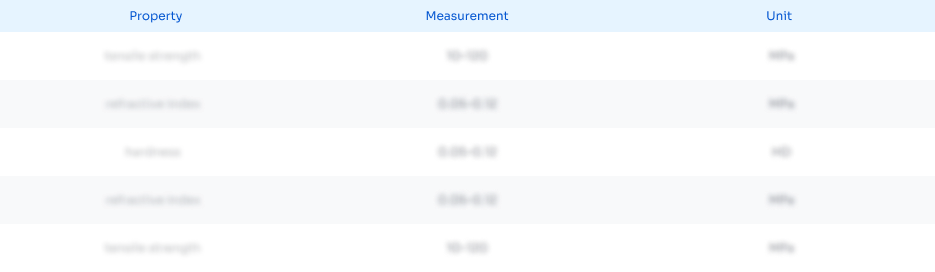
Abstract
Description
Claims
Application Information
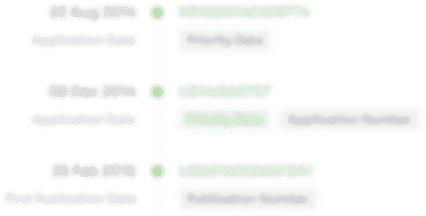
- R&D
- Intellectual Property
- Life Sciences
- Materials
- Tech Scout
- Unparalleled Data Quality
- Higher Quality Content
- 60% Fewer Hallucinations
Browse by: Latest US Patents, China's latest patents, Technical Efficacy Thesaurus, Application Domain, Technology Topic, Popular Technical Reports.
© 2025 PatSnap. All rights reserved.Legal|Privacy policy|Modern Slavery Act Transparency Statement|Sitemap|About US| Contact US: help@patsnap.com