Traffic flow prediction method fusing space-time attention neural network and traffic model
A technology of traffic model and neural network, applied in the field of traffic flow prediction that integrates spatio-temporal attention neural network and traffic model, it can solve the problem that the speed-flow model ignores the spatial structure of the road network and cannot simultaneously capture the temporal correlation and spatial correlation of data And other issues
- Summary
- Abstract
- Description
- Claims
- Application Information
AI Technical Summary
Problems solved by technology
Method used
Image
Examples
Embodiment Construction
[0027] In order to better understand the technical solutions of the present invention, the embodiments of the present invention will be described in detail below in conjunction with the accompanying drawings. It should be clear that the described embodiments and all other embodiments obtained by persons of ordinary skill in the art without creative efforts fall within the protection scope of the present invention.
[0028] refer to figure 1 , a traffic flow forecasting method that fuses a spatiotemporal attention neural network and a traffic model (Greenshields parabolic model), comprising the following steps:
[0029] Step 101, input the characteristic data and adjacency matrix of the traffic road network. Input the flow data and speed data of the road network respectively;
[0030] In step 102, the input feature data is divided into time slices, and GAT characterization is performed on each time slice to obtain a new representation of node features. The GAT operation is p...
PUM
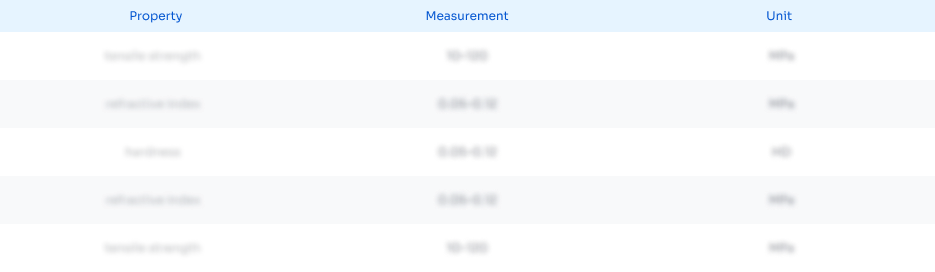
Abstract
Description
Claims
Application Information
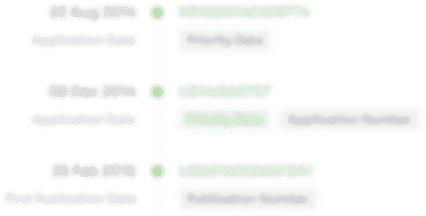
- R&D
- Intellectual Property
- Life Sciences
- Materials
- Tech Scout
- Unparalleled Data Quality
- Higher Quality Content
- 60% Fewer Hallucinations
Browse by: Latest US Patents, China's latest patents, Technical Efficacy Thesaurus, Application Domain, Technology Topic, Popular Technical Reports.
© 2025 PatSnap. All rights reserved.Legal|Privacy policy|Modern Slavery Act Transparency Statement|Sitemap|About US| Contact US: help@patsnap.com