Unsupervised two-stage field adaptive method
An adaptive and unsupervised technology, applied in neural learning methods, instruments, biological neural network models, etc., can solve problems such as reducing the distribution difference between domains, achieve high classification accuracy, improve performance, and achieve the effect of effective knowledge transfer
- Summary
- Abstract
- Description
- Claims
- Application Information
AI Technical Summary
Problems solved by technology
Method used
Image
Examples
Embodiment Construction
[0010] The present invention proposes an unsupervised two-stage domain adaptive method, which realizes the alignment between the source domain and the target domain on four levels, and reduces distribution differences. They are: subspace alignment, data alignment, label alignment, and graph structure alignment.
[0011] Subspace Alignment Item: P s and P t Defined as the source domain subspace projection and the target domain subspace projection respectively, minimizing the distance between the projections of two specific domains in the manifold space can reduce the domain shift. The subspace alignment term is defined as follows:
[0012]
[0013] Data alignment term: In order to narrow the distribution difference between the source domain and the target domain, an optimal target projection P is learned by using the intrinsic information of the data t . The target data is assumed to be linearly represented by the source domain data in a common subspace, and by imposing ...
PUM
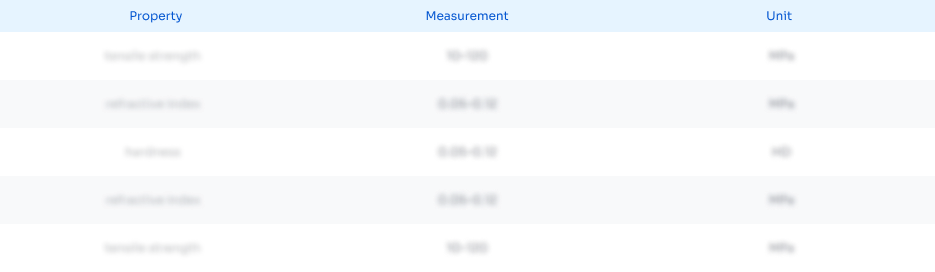
Abstract
Description
Claims
Application Information
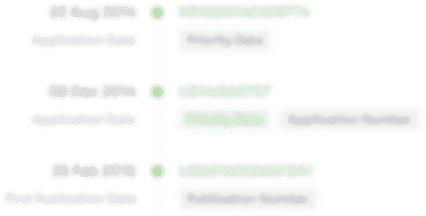
- R&D
- Intellectual Property
- Life Sciences
- Materials
- Tech Scout
- Unparalleled Data Quality
- Higher Quality Content
- 60% Fewer Hallucinations
Browse by: Latest US Patents, China's latest patents, Technical Efficacy Thesaurus, Application Domain, Technology Topic, Popular Technical Reports.
© 2025 PatSnap. All rights reserved.Legal|Privacy policy|Modern Slavery Act Transparency Statement|Sitemap|About US| Contact US: help@patsnap.com