Deep heterogeneous graph embedding model based on feature fusion
A feature fusion and graph embedding technology, applied in the deep heterogeneous graph embedding field of the deep heterogeneous graph embedding model, can solve the problems of lack of careful design of deep structure and insufficient expression of features at different levels.
- Summary
- Abstract
- Description
- Claims
- Application Information
AI Technical Summary
Problems solved by technology
Method used
Image
Examples
Embodiment Construction
[0025] The present invention will be described in further detail below in conjunction with the accompanying drawings and specific embodiments. It should be understood that the specific embodiments described here are only used to explain the present invention, not to limit the present invention.
[0026] The present invention is a graph embedding method of a deep heterogeneous graph embedding model based on feature fusion. The overall structure of the model is as follows: figure 1 As shown, the graph embedding process of the model includes meta-path subgraph extraction, residual graph attention node embedding, inter-layer feature fusion, semantic feature fusion and node category prediction.
[0027] First, the present invention is implemented using three heterogeneous graph networks constructed from real data, namely the citation network ACM, the citation network DBLP, and the business network IMDB. The specific information of the datasets is shown in Table 1.
[0028]
[0...
PUM
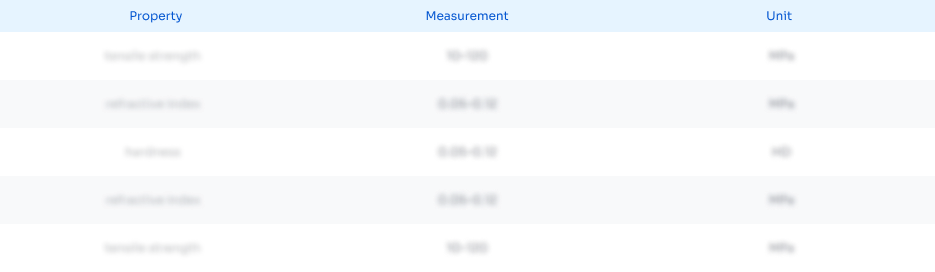
Abstract
Description
Claims
Application Information
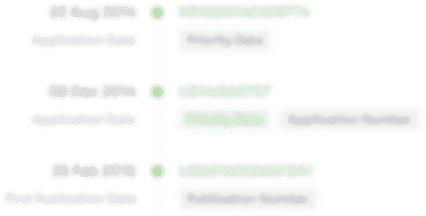
- R&D
- Intellectual Property
- Life Sciences
- Materials
- Tech Scout
- Unparalleled Data Quality
- Higher Quality Content
- 60% Fewer Hallucinations
Browse by: Latest US Patents, China's latest patents, Technical Efficacy Thesaurus, Application Domain, Technology Topic, Popular Technical Reports.
© 2025 PatSnap. All rights reserved.Legal|Privacy policy|Modern Slavery Act Transparency Statement|Sitemap|About US| Contact US: help@patsnap.com