Bayesian tensor completion algorithm based on complex noise
A noise and completion technology, applied in the field of tensor completion, can solve the problems of overfitting, not considering missing data, unable to deal with complex noise, etc., to achieve accurate completion and denoising, good completion and denoising. Effect
- Summary
- Abstract
- Description
- Claims
- Application Information
AI Technical Summary
Problems solved by technology
Method used
Image
Examples
Embodiment Construction
[0058] In order to make the technical means, creative features, goals and effects realized by the present invention easy to understand, the following describes the complex noise-based Bayesian tensor completion algorithm of the present invention in detail with reference to the embodiments and the accompanying drawings.
[0059]
[0060] This embodiment provides a Bayesian tensor completion algorithm based on complex noise (MoG BTC-CP), which is used to represent target data with missing measurement values as corresponding tensors, and to complete the tensors at the same time and denoising to obtain a more accurate tensor estimate, and interpolate the target data based on the tensor estimate, so as to complete and denoise the target data. figure 2 is a schematic diagram of a Bayesian network, such as figure 2 As shown, the completion method of this embodiment combines the framework of CP decomposition and Bayesian method to perform Gibbs sampling, and outputs the estimate...
PUM
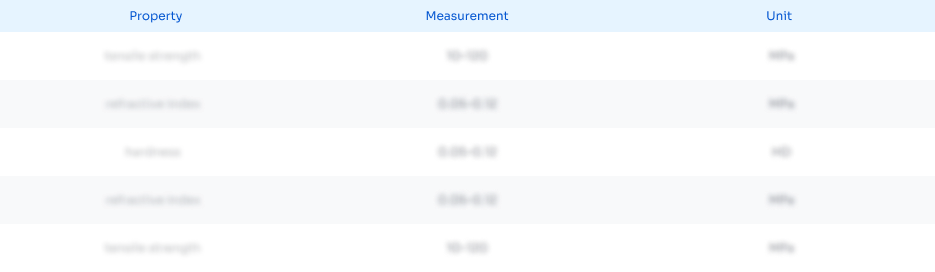
Abstract
Description
Claims
Application Information
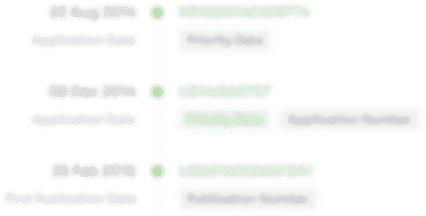
- R&D Engineer
- R&D Manager
- IP Professional
- Industry Leading Data Capabilities
- Powerful AI technology
- Patent DNA Extraction
Browse by: Latest US Patents, China's latest patents, Technical Efficacy Thesaurus, Application Domain, Technology Topic, Popular Technical Reports.
© 2024 PatSnap. All rights reserved.Legal|Privacy policy|Modern Slavery Act Transparency Statement|Sitemap|About US| Contact US: help@patsnap.com