Federal machine learning method and server
A machine learning and machine learning model technology, applied in neural learning methods, ensemble learning, biological neural network models, etc., can solve problems such as inaccuracy and unreliability
- Summary
- Abstract
- Description
- Claims
- Application Information
AI Technical Summary
Problems solved by technology
Method used
Image
Examples
Embodiment Construction
[0030] Various embodiments of the present invention provide a federated machine learning method and a server thereof.
[0031] As described in the Background, recent work has developed decentralized federated learning approaches that can train deep learning models across multiple data sources without sharing sensitive information. These existing federated learning approaches have proven successful, but may still suffer from inaccuracy and / or unreliability, depending on the data sources on which they are trained. In particular, according to various embodiments of the present invention, it can be determined that these existing federated learning approaches either assume that each of the multiple data sources provides the same quality of data (labeled data), or do not take into account the multiple data Different quality of data between sources, resulting in inaccuracy and / or unreliability.
[0032] For example and without limitation, according to various embodiments, it is note...
PUM
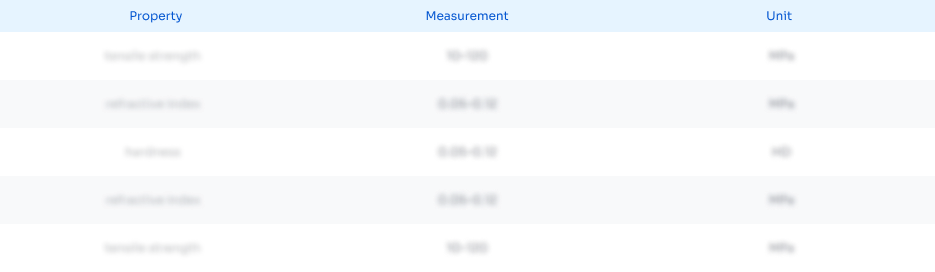
Abstract
Description
Claims
Application Information
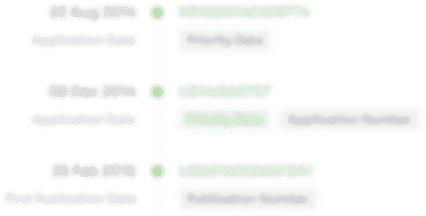
- R&D Engineer
- R&D Manager
- IP Professional
- Industry Leading Data Capabilities
- Powerful AI technology
- Patent DNA Extraction
Browse by: Latest US Patents, China's latest patents, Technical Efficacy Thesaurus, Application Domain, Technology Topic.
© 2024 PatSnap. All rights reserved.Legal|Privacy policy|Modern Slavery Act Transparency Statement|Sitemap