Image classification method based on linear self-attention Transform
A classification method and attention technology, applied in the field of computer vision, can solve problems such as large amount of calculation, lack of translation invariance and locality, and achieve the effect of reducing computational complexity, reducing dependence, and improving network performance
- Summary
- Abstract
- Description
- Claims
- Application Information
AI Technical Summary
Problems solved by technology
Method used
Image
Examples
Embodiment Construction
[0031] The present invention is described in detail below in conjunction with the accompanying drawings for further explanation, so that those skilled in the art can more deeply understand the present invention and can implement it, but the following examples are only used to explain the present invention, not as the present invention limit.
[0032] like figure 1 As shown, an image classification method based on linear self-attention Transformer proposed by the present invention, the main body of the network model is composed of four different stages, and each stage is composed of overlapping convolutional coding module and Transformer module. The computational complexity of the attention mechanism of the Transformer module is linearly related to the number of input tokens. Compared with the ViT model and some of its variants, the computational complexity of the network model of the present invention is significantly reduced. Information is modeled, the present invention use...
PUM
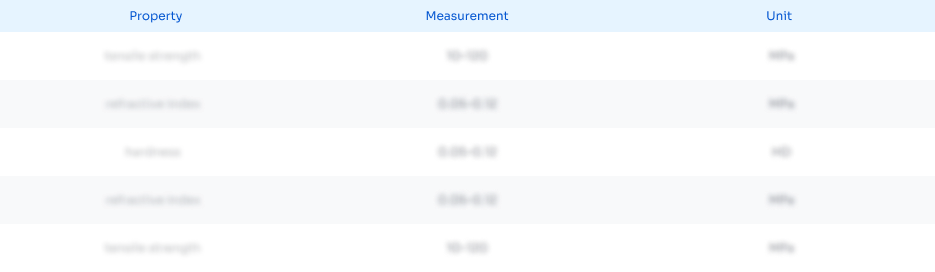
Abstract
Description
Claims
Application Information
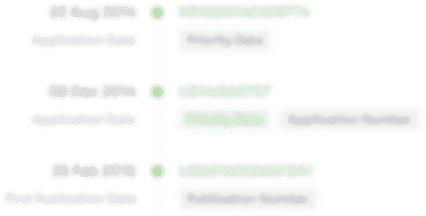
- R&D Engineer
- R&D Manager
- IP Professional
- Industry Leading Data Capabilities
- Powerful AI technology
- Patent DNA Extraction
Browse by: Latest US Patents, China's latest patents, Technical Efficacy Thesaurus, Application Domain, Technology Topic, Popular Technical Reports.
© 2024 PatSnap. All rights reserved.Legal|Privacy policy|Modern Slavery Act Transparency Statement|Sitemap|About US| Contact US: help@patsnap.com