Mechanical fault diagnosis method and system based on federal domain generalization
A technology for mechanical faults and diagnosis methods, applied in neural learning methods, machine learning, computer parts and other directions, can solve problems such as personalized training of models that do not consider the availability of target domain data, and achieve cross-domain fault diagnosis problems and reduce Differences, data security and the effect of improving the correct rate of fault diagnosis
- Summary
- Abstract
- Description
- Claims
- Application Information
AI Technical Summary
Problems solved by technology
Method used
Image
Examples
Embodiment 1
[0048] Let N clients contain N source domain datasets , the N+1th client has the target domain dataset ,in, represents the total number of samples in the target domain dataset, means the first A sample of the target domain dataset, The value range is from 1 to integers between. means the first source domain dataset samples, The value of is an integer between 1 and N. The target domain dataset does not participate in model training and is only used for testing, and the source domain participates in training.
[0049] There is a feature extraction network and a classification network in each client. Let the model set of N feature extraction networks be , the model set of N classification networks is . The global feature extraction network model in the central server is set as , the global classification network model is .
[0050] In order to effectively aggregate and use data on the premise of ensuring the data security of different clients and solv...
Embodiment 2
[0093] The second embodiment of the present disclosure provides a mechanical fault diagnosis system based on federal domain generalization, including:
[0094] A central server and a client, the central server is used to initialize the global model, the central server includes a global feature extraction network and a global classification network, and the central server simultaneously exchanges information with multiple clients; each Each client contains a feature extraction network and a classification network. It is assumed that N clients contain N source domain datasets, and the N+1th client contains target domain datasets.
PUM
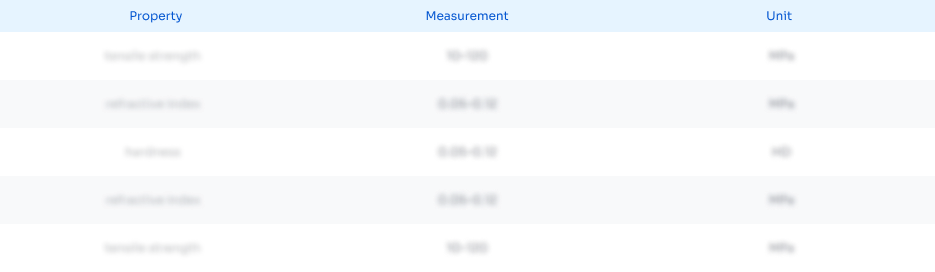
Abstract
Description
Claims
Application Information
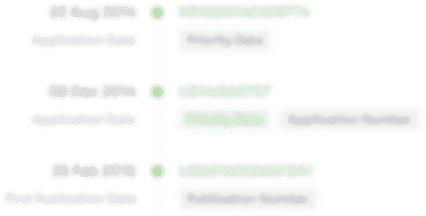
- R&D Engineer
- R&D Manager
- IP Professional
- Industry Leading Data Capabilities
- Powerful AI technology
- Patent DNA Extraction
Browse by: Latest US Patents, China's latest patents, Technical Efficacy Thesaurus, Application Domain, Technology Topic.
© 2024 PatSnap. All rights reserved.Legal|Privacy policy|Modern Slavery Act Transparency Statement|Sitemap