Multi-label image classification method based on direct push type semi-supervised deep learning
A technology of deep learning and classification methods, applied in neural learning methods, character and pattern recognition, instruments, etc., to achieve good robustness and reduce labeling costs
- Summary
- Abstract
- Description
- Claims
- Application Information
AI Technical Summary
Problems solved by technology
Method used
Image
Examples
Embodiment
[0117] Based on two currently commonly used deep convolutional neural network models, AlexNet and GoogLeNet, experimental evaluations are performed on two multi-label image classification datasets, Pascal VOC 2007 and MIR Flickr 25K, to fully verify the effectiveness of the method of the present invention.
[0118] PascalVOC2007 dataset: This dataset contains 9963 images in 20 classes. Each image has 1 to 5 class labels, with an average of 1.8 class labels. The official standard data division and evaluation indicators are used, that is, 5011 images in the training set and 4952 images in the test set, and the evaluation indicator is mAP (mean of Average Precision). The mAP value ranges from 0 to 1. The larger the mAP value, the better the classification performance.
[0119] MIR Flickr 25K dataset: This dataset contains 25000 images, which are annotated into 24 classes. For 14 of them, image sets with more prominent corresponding class instances are selected as a new class. ...
PUM
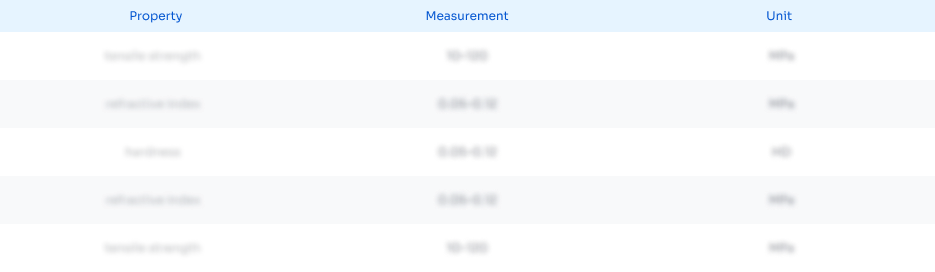
Abstract
Description
Claims
Application Information
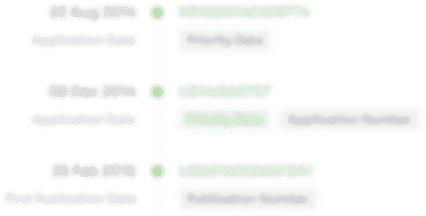
- R&D
- Intellectual Property
- Life Sciences
- Materials
- Tech Scout
- Unparalleled Data Quality
- Higher Quality Content
- 60% Fewer Hallucinations
Browse by: Latest US Patents, China's latest patents, Technical Efficacy Thesaurus, Application Domain, Technology Topic, Popular Technical Reports.
© 2025 PatSnap. All rights reserved.Legal|Privacy policy|Modern Slavery Act Transparency Statement|Sitemap|About US| Contact US: help@patsnap.com