Deep network waveform synthesis method and device based on filter bank frequency discrimination
A filter bank and deep network technology, applied in speech synthesis, instrumentation, speech analysis, etc., can solve problems such as aliasing failure in high-frequency parts, spectral distortion in high-frequency bands, large size, etc., to reduce spectral distortion and infer speed Improve the effect of clearing the details of the mel spectrum
- Summary
- Abstract
- Description
- Claims
- Application Information
AI Technical Summary
Problems solved by technology
Method used
Image
Examples
Embodiment 1
[0062] Examples of the present invention provide a method of deep network text transposition voice waveform synthesis based on the frequency distinction of filters. See Figure 1-Figure 6 This method includes the following steps:
[0063] 101: The voice data set used by the training center, the transcription text corresponding to the voice, and the test text, to give the front -end network of acoustic model from text to Melphen;
[0064] 102: Divide the voice set of the data into the training set, and then calculate the Mel spectrum of each voice in turn, thereby constructing the training Merr spectrum data set to achieve the pre -processing of the data set;
[0065] 103: Build the network:
[0066] Build figure 1 The generator network shown, including: Transposed Convolutional module and more multi-receptive filed fusion (MRF); and figure 2 The multi -frequency discriminator network shown is composed of a number of sub -identifier, and each sub -identifier is processed to the signal...
Embodiment 2
[0080] The following combined with specific calculation formulas and instances, the scheme in Example 1 is further introduced. For details, please refer to the description below:
[0081] 1. Based on generating a vocal coder design that generates confrontation network
[0082] 1. Network structure
[0083] Suppose it is in low -dimensional space There is a simple and easy -to -sample distribution P (Z), and P (Z) is usually a standard diverse normal distribution n (0, i). Construct a mapping function with a neural network Known as the generation network. Using the powerful fitting ability of the neural network, G (Z) obey data distribution P r (X). This model is called implicit density model. The so -called implicit density model refers r (X), but the modeling process.
[0084] One key to implicit density model is how to ensure that the sample generated by generating networks must be obediently obediently distributed.
[0085] Generating a confrontation network is to obey the re...
Embodiment 3
[0150] The sampling rate of the audio used in the experiment is 22.05kHz, and the frequency sampling vector length is n = 512. Take the ninth filter in the filter group as an example. L F H ) = [700Hz, 1000Hz], F L = 700Hz, F H = 1000Hz, F s = 22050Hz, N = 512 Interture (19), get P = 16, Q = 8, and further convulsure the Hanying window with a length of N and the length of the flip of N Window accumulation element W c (N), substitute the above value (25), you can bring the filter coefficient g (n), and further find the frequency response function G (j2πf) of the filter, such as Image 6 Show in the black line. Take the fourth filter in the filter group as an example, Figure 7 List the original voice wave shape and its spectrum, and the waveform and spectrum after being filtered by Analytic Filter 4.
[0151] Secondly, the overall effect of the embodiments of the invention in the end -to -end of the model is verified. First, a TTS front -end model is used to generate the intermediate...
PUM
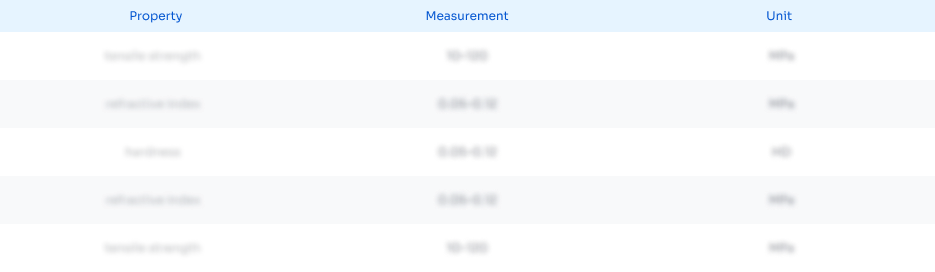
Abstract
Description
Claims
Application Information
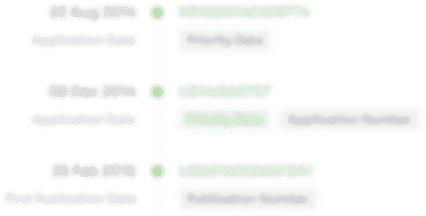
- R&D Engineer
- R&D Manager
- IP Professional
- Industry Leading Data Capabilities
- Powerful AI technology
- Patent DNA Extraction
Browse by: Latest US Patents, China's latest patents, Technical Efficacy Thesaurus, Application Domain, Technology Topic, Popular Technical Reports.
© 2024 PatSnap. All rights reserved.Legal|Privacy policy|Modern Slavery Act Transparency Statement|Sitemap|About US| Contact US: help@patsnap.com