Medical image recognizing method
A medical image and normalization technology, applied in the field of medical image recognition, can solve the problems of general adaptability, high dimensionality, and impracticality of knowledge mining applications that do not have other organs
- Summary
- Abstract
- Description
- Claims
- Application Information
AI Technical Summary
Problems solved by technology
Method used
Image
Examples
Embodiment Construction
[0080] Taking liver CT medical images as an example, the execution process of the present invention will be briefly described below. In this example, a total of 120 liver CT images were selected, including 80 normal images and 40 abnormal images. The specific execution steps are as follows:
[0081] like figure 1 Shown, a kind of method for medical image recognition comprises the construction of association rule classification database and its update and medical image recognition step, it is characterized in that, the construction of described association rule classification database and its update step include the following steps:
[0082] (1) Perform format conversion and medical image denoising and enhancement processing on the 120 liver CT images respectively.
[0083] (2) Extract the relevant features of each image and perform normalization processing, the results are shown in Table 1. The features extracted by the present invention include mean value, variance, slope, ...
PUM
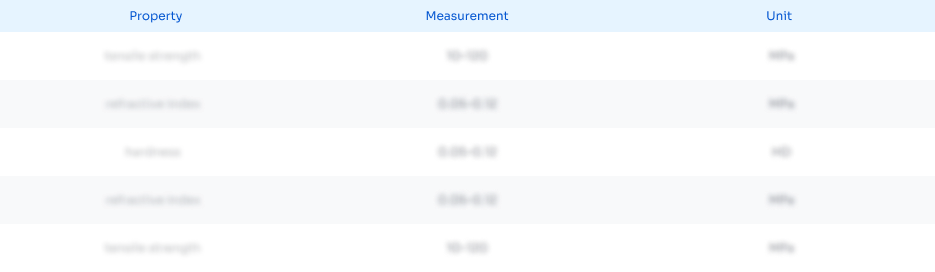
Abstract
Description
Claims
Application Information
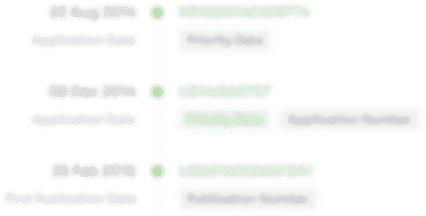
- R&D Engineer
- R&D Manager
- IP Professional
- Industry Leading Data Capabilities
- Powerful AI technology
- Patent DNA Extraction
Browse by: Latest US Patents, China's latest patents, Technical Efficacy Thesaurus, Application Domain, Technology Topic, Popular Technical Reports.
© 2024 PatSnap. All rights reserved.Legal|Privacy policy|Modern Slavery Act Transparency Statement|Sitemap|About US| Contact US: help@patsnap.com