Method for synthesizing three-dimensional human body movement based on non-linearity manifold study
A nonlinear manifold and human motion technology, applied in animation production, 3D image processing, image data processing, etc., can solve problems such as loose distribution of data samples and inability to achieve precise control of synthetic motion semantics
- Summary
- Abstract
- Description
- Claims
- Application Information
AI Technical Summary
Problems solved by technology
Method used
Image
Examples
Embodiment Construction
[0047] The 3D human motion synthesis method based on nonlinear manifold learning includes the following steps:
[0048] 1) Expand a set of preprocessed 3D human motion data with the same length into a set of vectors, which are used as the input of the nonlinear manifold learning method, and map this set of sparse 3D human motion samples through the nonlinear manifold learning method Construct motion semantic parameter space on low-dimensional manifold;
[0049] 2) Perform dense resampling with uniform distribution on the low-dimensional motion semantic parameter space, and apply the resampling coefficient set to the sparsely distributed motion samples in the original motion space to obtain dense and uniform motion samples in the high-dimensional space;
[0050] 3) The high-dimensional motion samples obtained by dense resampling are remapped through nonlinear manifold learning to obtain the final low-dimensional motion semantic parameter space;
[0051] 4) The user selects the...
PUM
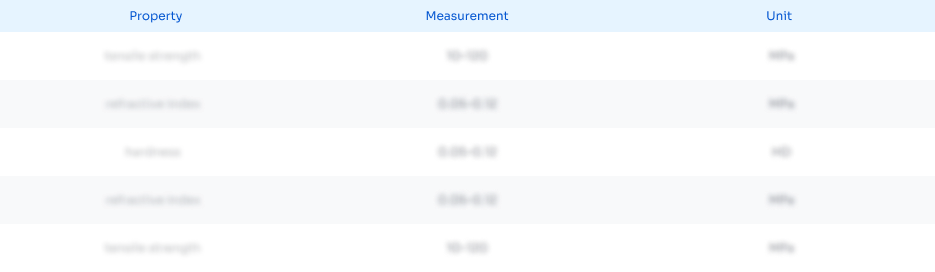
Abstract
Description
Claims
Application Information
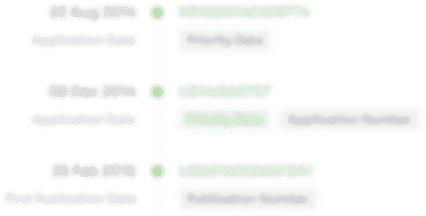
- R&D Engineer
- R&D Manager
- IP Professional
- Industry Leading Data Capabilities
- Powerful AI technology
- Patent DNA Extraction
Browse by: Latest US Patents, China's latest patents, Technical Efficacy Thesaurus, Application Domain, Technology Topic, Popular Technical Reports.
© 2024 PatSnap. All rights reserved.Legal|Privacy policy|Modern Slavery Act Transparency Statement|Sitemap|About US| Contact US: help@patsnap.com