Target predicting and tracking method based on probability graph model
A probabilistic graph model and target tracking technology, which can be used in measuring devices, image analysis, image data processing, etc., can solve problems such as the difficulty of EKF algorithm
- Summary
- Abstract
- Description
- Claims
- Application Information
AI Technical Summary
Problems solved by technology
Method used
Image
Examples
Embodiment
[0037] Probabilistic graphical models:
[0038] An undirected graph model is expressed as G=(V, E), where V represents a set of vertices (nodes), and E represents a set of edges (edges). Such as figure 1 As shown, each vertex s∈V represents a random variable x s , s∈V, the relationship between variables can be represented by the graph model structure. The variables represented by the undirected graph are considered to be discrete, and these variables x are Markov random variables related to the graph structure, and its distribution p(x) is expressed as:
[0039] p(x)=κ∏ s∈V Ψ s (x s )∏ (s,t)∈E Ψ st (x s , x t )
[0040] where κ is a normalization constant: Ψ s (x s ) is a vertex-compatible function that depends on the variable x s ; st (x s , x t ) is an edge compatible function, which depends on the variable x s and x t The connecting line (s, t). In general, the random variable x is an implicit variable to be sought and cannot be observed. Assume that the...
PUM
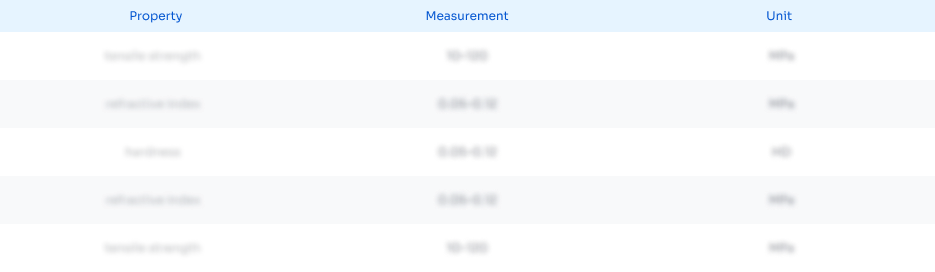
Abstract
Description
Claims
Application Information
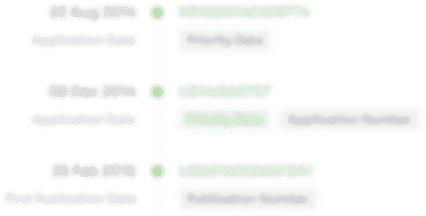
- R&D Engineer
- R&D Manager
- IP Professional
- Industry Leading Data Capabilities
- Powerful AI technology
- Patent DNA Extraction
Browse by: Latest US Patents, China's latest patents, Technical Efficacy Thesaurus, Application Domain, Technology Topic, Popular Technical Reports.
© 2024 PatSnap. All rights reserved.Legal|Privacy policy|Modern Slavery Act Transparency Statement|Sitemap|About US| Contact US: help@patsnap.com